Applications of Machine Learning: From Theory to Reality
Explore practical applications of machine learning in this comprehensive guide. From theory to reality, discover real-world examples shaping the future of technology.
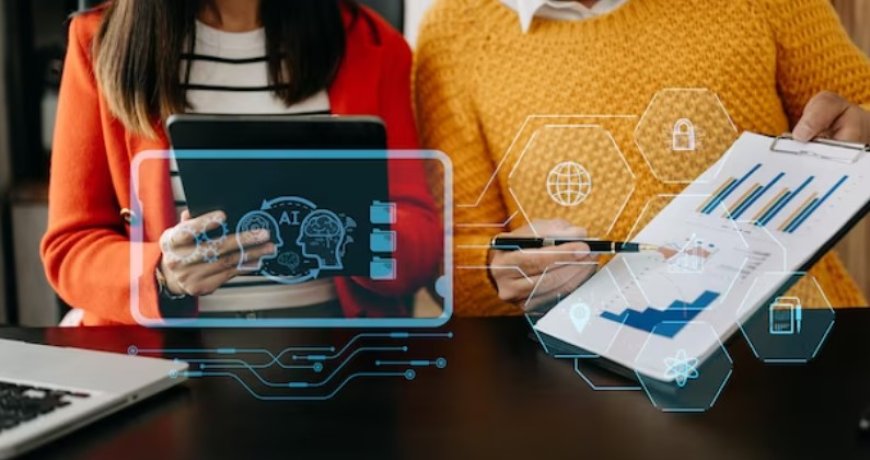
Machine Learning (ML) is like the brains behind the scenes, making our digital world smarter. Consider it an intelligent and experienced technical companion. In short, ML is a branch of artificial intelligence where computer systems are designed to learn and improve without specific programming. Now, why is it gaining so much attention? Imagine having a personal assistant that anticipates your needs – that's ML at work. It analyzes data, recognizes patterns, and makes predictions, allowing machines to make decisions independently.
ML isn't just a trendy word; it's transforming industries. From healthcare to finance, ML is enhancing efficiency and accuracy. In healthcare, it helps predict diseases, while in finance, it optimizes investment strategies. The more data ML processes, the smarter it becomes.ML has become an essential tool as organizations generate enormous amounts of data. These days, the focus is on gaining insights that will influence the future rather than just analyzing data. So grab a seat, and let's explore the world of machine learning (ML), where computers are more than just tools they're smart friends who can make our lives easier.
Understanding the foundational concepts of Machine Learning
Machine Learning, at its core, enables computers to learn and make decisions independently, without specific programming. Let's break down the fundamental aspects of this fascinating field. What exactly is Machine Learning? It's a subset of artificial intelligence that enables systems to learn and improve based on experience. There are three main types: supervised learning, unsupervised learning (letting the model explore patterns in unlabeled data), and reinforcement learning (teaching the model by rewarding desired behaviors).
A brief historical overview reveals that Machine Learning's origins can be traced back to the mid-20th century, gaining prominence with the convergence of powerful computing and substantial datasets. Key milestones include the development of neural networks and breakthroughs in algorithmic efficiency.
Challenges in applying theoretical machine learning concepts to real-world situations
Overcoming the Gap between Academia and Industry:
One significant challenge is aligning theoretical advancements in ML, often originating in academic settings, with the practical needs of industries. Academic research may prioritize innovation and theoretical rigor but may need more direct relevance to the practical challenges faced by industries. Successful implementation requires collaboration between academia and industry to ensure theoretical advancements align with real-world applicability.
Addressing Misconceptions about ML Capabilities:
Another hurdle involves dispelling misconceptions about the capabilities of ML. While theoretical frameworks may suggest broad possibilities, practical implementation faces limitations such as data quality, ethical considerations, and algorithmic bias. Managing expectations and communicating the actual potential and constraints of ML technology to stakeholders is crucial. This requires a nuanced understanding of both theoretical foundations and practical constraints.
Importance of Interdisciplinary Collaboration:
An important factor in overcoming these challenges is developing interdisciplinary collaboration. ML implementations require expertise not only in machine learning algorithms but also in domain-specific knowledge. Collaborations between data scientists, domain experts, ethicists, and other stakeholders enhance the development and deployment of ML applications. This interdisciplinary approach ensures a comprehensive understanding of the problem and promotes the creation of theoretically sound and practically effective solutions.
How Can Machine Learning Be Applied in Real-World Scenarios?
Introducing the Real-world Applications of Machine Learning
Healthcare Applications:
Predictive Analytics in Disease Prevention: By analyzing patient data, machine learning can be used to identify patterns that indicate the possibility of developing certain diseases. This aids in proactive disease prevention and personalized healthcare.
Enhancing Medical Imaging through ML Algorithms: ML algorithms enhance the accuracy of medical imaging, facilitating quicker and more precise diagnosis. This includes image recognition and segmentation for conditions such as tumors.
Drug Discovery and Development with Machine Learning: ML accelerates drug discovery by analyzing large datasets to identify potential drug candidates, predict their efficacy, and optimize drug development processes.
Finance Applications:
Fraud Detection and Prevention Using ML Models: ML models are employed to detect unusual patterns and anomalies in financial transactions, helping to prevent fraudulent activities in real-time.
Risk Management through Predictive Analytics: Predictive analytics powered by ML assesses financial risks by analyzing historical data and market trends, enabling institutions to make informed decisions to mitigate potential risks.
Algorithmic Trading Strategies Powered by Machine Learning: ML algorithms analyze market data to identify trading patterns and trends, optimizing investment strategies for better returns and risk management.
Marketing Applications:
Customer Behavior Analysis for Targeted Marketing:
ML analyzes customer behavior data to understand preferences and predict future actions. This insight is used to personalize marketing strategies for better customer engagement and conversion.
Sentiment Analysis and Social Media Marketing:
ML-driven sentiment analysis gauges public opinion on social media, helping businesses understand customer sentiments and adapt marketing strategies accordingly.
Trend Prediction and Demand Forecasting through ML:
ML algorithms analyze market trends, historical data, and external factors to predict future trends and forecast demand. This aids in inventory management and ensures timely response to market changes.
Ethical Considerations in ML Applications:
Bias and Fairness
The challenge of bias in ML algorithms:
One big challenge in machine learning (ML) is tackling bias. Bias can unintentionally find its way into ML algorithms, leading to unfair outcomes. To counter this, it's crucial to implement strategies that promote fairness and eliminate bias in ML applications.
Strategies for Creating Fair and Unbiased ML Applications:
Diverse and Representative Data: Ensure that the training data is diverse and representative of the real-world population. This helps in mitigating biases that might emerge from skewed datasets.
Regular Audits and Assessments: Conduct regular audits of ML models to identify and rectify any bias that may have developed over time. This ongoing evaluation ensures the system remains fair and unbiased throughout its lifecycle.
Explainable AI (XAI): Implement transparent and explainable AI models that allow developers and users to understand how decisions are made. This transparency is essential for uncovering and addressing biases.
Transparency and Accountability in ML Decision-Making:
Importance of Understanding ML Decision-Making:
User Trust: Users are more likely to trust ML applications when they understand the decision-making process. This trust is important for widespread acceptance and adoption.
Identifying and Addressing Issues: Transparent models facilitate the identification of potential issues or biases. When the decision-making process is clear, developers can address concerns promptly.
Establishing Accountability in ML Model Development and Deployment:
Documentation and Traceability: Keep comprehensive documentation throughout the development process to ensure that decisions and choices are traceable. This documentation aids in accountability by providing a clear record of the model's evolution.
Ethics Committees and Review Boards: Establish ethics committees or review boards to provide an external perspective on the ethical implications of ML applications. This external input ensures a holistic consideration of ethical concerns.
In conclusion, the past year has seen Machine Learning (ML) make remarkable improvements from theory to practical application. Foundational concepts such as supervised, unsupervised, and reinforcement learning underscore its several methods. Bridging academia and industry remains a challenge, necessitating collaboration and alignment with real-world needs. Real-world applications, spanning healthcare, finance, and marketing, showcase ML's predictive and analytical capabilities. Challenges such as bias and ethical considerations require ongoing attention. Strategies like different data, regular audits, and transparent models are essential for creating fair and unbiased ML applications.