Data Quality Assurance: The Key to Reliable and Trustworthy Data Engineering
Learn how to ensure accuracy, consistency, and credibility in your data through effective quality assurance practices.
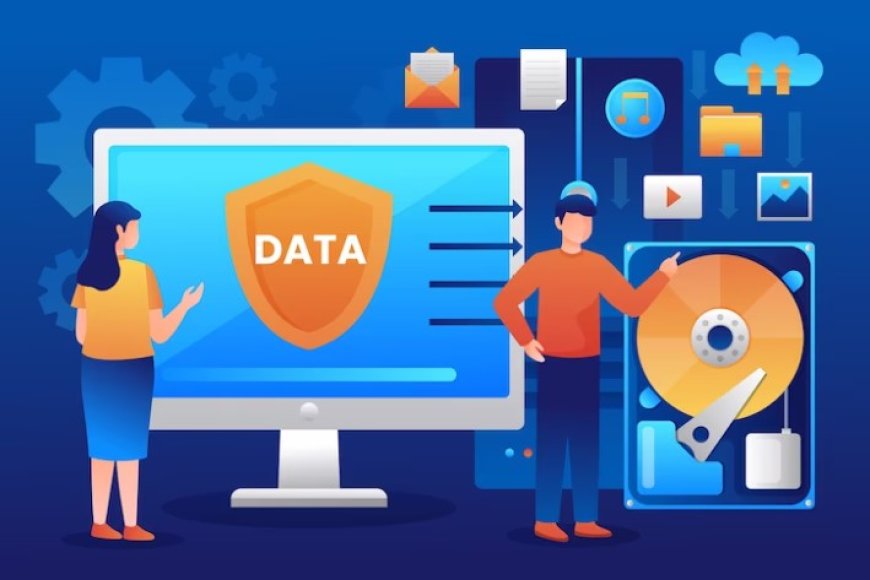
In today's data-driven landscape, the integrity of data is paramount. Data Quality Assurance serves as the cornerstone of reliable and trustworthy data engineering. Ensuring the accuracy, completeness, and consistency of data has far-reaching implications for informed decision-making, regulatory compliance, and the efficacy of analytical and machine learning endeavors. This outline explores the dimensions of data quality, its impact, strategies for assurance, collaborative efforts, and future trends, emphasizing its critical role in the data ecosystem.
Importance of Reliable and Trustworthy Data Engineering
-
Informed Decision-Making: Reliable data engineering ensures that the data used for decision-making is accurate and trustworthy, leading to better business strategies and outcomes.
-
Business Insights: Trustworthy data engineering enables meaningful insights, helping organizations understand customer behavior, market trends, and operational efficiencies accurately.
-
Regulatory Compliance: Data engineering that prioritizes reliability ensures adherence to data privacy regulations and prevents legal and financial liabilities.
-
Data-Driven Innovation: A solid foundation of trustworthy data allows for innovative data-driven initiatives, such as machine learning, AI applications, and predictive analytics.
-
Stakeholder Confidence: Consistently reliable data builds trust among stakeholders, including customers, investors, and partners.
-
Cost Efficiency: Data quality assurance reduces the costs associated with correcting errors and inconsistencies downstream in the data processing pipeline.
-
Efficient Operations: Accurate data engineering supports streamlined operations, minimizing disruptions caused by incorrect or incomplete data.
-
Effective Resource Allocation: Reliable data enables organizations to allocate resources effectively by basing decisions on accurate insights rather than guesswork.
-
Competitive Advantage: Organizations with reliable data engineering gain a competitive edge by making more informed decisions faster than their competitors.
-
Long-Term Viability: Sustainable growth and success hinge on a foundation of trustworthy data engineering, enabling organizations to adapt to evolving challenges.
Understanding Data Quality
Data quality forms the cornerstone of any successful data engineering endeavor. It refers to the accuracy, completeness, consistency, timeliness, and validity of data throughout its lifecycle. Accuracy ensures that data values reflect reality, while completeness guarantees that all necessary information is present. Consistency ensures uniformity across datasets, and timeliness ensures data relevance within its operational context. Validity verifies whether data adheres to predefined rules and standards.
The impact of poor data quality can be profound, extending beyond technical concerns to encompass business operations and decision-making processes. Inaccurate or incomplete data can lead to faulty insights, misguided strategic choices, and compromised customer experiences. As organizations increasingly rely on data-driven insights, data quality issues can erode trust in the data itself and undermine the effectiveness of downstream analytics, reporting, and machine learning initiatives. Recognizing and addressing these dimensions of data quality is essential for organizations to derive meaningful value from their data assets.
The Data Engineering Process
The data engineering process is a critical component of ensuring data quality and reliability throughout an organization's data lifecycle. This process encompasses several key stages that collectively contribute to the transformation of raw data into valuable insights.
Data Collection and Ingestion: The journey begins with the collection and ingestion of data from various sources, such as databases, APIs, logs, and external feeds. During this phase, data engineers must meticulously design pipelines that can extract data efficiently while maintaining its integrity. Challenges related to data quality can arise at this stage, including data inconsistencies, missing values, and inaccuracies originating from the source.
Data Transformation and Cleaning: Once collected, the data often requires preprocessing to make it suitable for analysis. This involves transformations such as normalization, standardization, and data cleaning to eliminate inconsistencies and inaccuracies. Identifying and addressing outliers and missing values are crucial steps in this stage to prevent skewed insights and unreliable analyses.
Data Storage and Integration: Properly storing and integrating data is paramount for maintaining data quality. Data engineers need to design robust database schemas that reflect the underlying business logic and relationships. Integrating data from multiple sources introduces challenges related to data mapping, schema alignment, and data format compatibility, which can impact data quality if not handled appropriately.
Importance of Data Quality Assurance
Data Quality Assurance is of paramount importance in today's data-driven world. It serves as the foundation for reliable and effective decision-making across various sectors and industries. Ensuring the accuracy, completeness, consistency, and reliability of data is essential to extract meaningful insights and make informed choices. High-quality data minimizes the risk of erroneous conclusions and faulty strategies that could stem from flawed information. It enhances the overall efficiency of operations, boosts customer satisfaction, and fosters innovation. Moreover, data compliance and regulatory requirements demand meticulous data quality, further underscoring its significance. By investing in robust data quality assurance processes, organizations can maintain their competitive edge, build trust with stakeholders, and harness the full potential of their data resources.
Data Quality Assurance Strategies
Data Quality Assurance (DQA) strategies are essential components of any data-driven organization's operations. These strategies encompass a range of systematic processes and practices designed to ensure that the data collected, processed, and analyzed meet predefined standards of accuracy, completeness, reliability, and consistency. A robust DQA strategy involves various stages, starting with data collection, where meticulous protocols are established to capture information accurately and comprehensively. This is followed by thorough data validation and cleansing, involving checks for errors, duplicates, and outliers, thus enhancing the overall integrity of the dataset.
Another crucial facet of DQA is the establishment of data governance policies and frameworks. These policies define roles, responsibilities, and procedures for data management, ensuring that data is treated as a valuable organizational asset. Regular audits and reviews are performed to identify any deviations from the established quality standards and to take corrective actions promptly.
Advanced technologies like machine learning algorithms and automated tools play a pivotal role in DQA strategies. These technologies can help in identifying patterns, anomalies, and potential data quality issues that might go unnoticed through manual inspection. Moreover, continuous monitoring and real-time alerts contribute to maintaining data quality over time.
Implementing Data Quality Frameworks
Implementing Data Quality Frameworks is crucial for organizations aiming to harness the full potential of their data assets. A Data Quality Framework provides a structured approach to assess, improve, and maintain the quality of data throughout its lifecycle. This involves defining clear data quality metrics and standards, establishing processes to monitor and measure data quality, identifying sources of data errors or inconsistencies, and implementing corrective actions. Such frameworks enable businesses to make well-informed decisions, enhance customer experiences, and ensure regulatory compliance. By incorporating data quality as a strategic priority, organizations can enhance their data-driven initiatives, foster trust among stakeholders, and ultimately achieve better outcomes in an increasingly data-centric world.
Collaborative Data Quality Efforts
Collaborative data quality efforts are essential in today's data-driven landscape, where accurate and reliable information is paramount for informed decision-making and successful business operations. Such endeavors involve the coordinated actions of various stakeholders across an organization, aiming to ensure that data is accurate, consistent, complete, and up-to-date. These efforts typically encompass data profiling, cleansing, validation, and monitoring, with teams from different departments working together to identify and rectify errors, inconsistencies, and redundancies within the data. Through shared expertise, resources, and tools, organizations can establish robust data quality frameworks that enhance overall data integrity, boost confidence in analytics, and support strategic initiatives. Effective collaboration not only fosters a culture of data responsibility but also drives better insights, operational efficiency, and ultimately, improved outcomes across the board.
Future Trends in Data Quality Assurance
In the ever-evolving landscape of data management, several prominent trends are shaping the future of data quality assurance. These trends are driven by the growing complexity of data ecosystems, the increasing reliance on data-driven decision-making, and the need to ensure accuracy, reliability, and compliance in a rapidly changing technological and regulatory environment.
Advancements in AI and Automation:
One of the most significant trends in data quality assurance is the increasing reliance on artificial intelligence (AI) and automation. AI-powered tools and algorithms are becoming instrumental in identifying, correcting, and preventing data quality issues. Machine learning models can learn from historical data patterns and automatically detect anomalies, inconsistencies, and inaccuracies within datasets. Automation streamlines the data quality process, reducing the manual effort required for data validation, cleansing, and enrichment. As AI continues to mature, it's expected that these technologies will play a pivotal role in maintaining high-quality data throughout its lifecycle.
Integration of Data Quality in DevOps Practices:
The integration of data quality practices into DevOps methodologies is gaining traction as organizations recognize the importance of ensuring data integrity throughout the development and deployment pipeline. Traditionally associated with software development, DevOps emphasizes collaboration and automation between development and IT operations teams. By incorporating data quality checks into the continuous integration and continuous delivery (CI/CD) process, organizations can identify and rectify data issues earlier in the development lifecycle. This integration not only reduces the risk of data defects making their way into production but also fosters a culture of shared responsibility for data quality.
Evolving Data Privacy Regulations and Their Impact on Data Quality:
The increasingly stringent data privacy regulations, such as the General Data Protection Regulation (GDPR) and the California Consumer Privacy Act (CCPA), have a profound impact on data quality practices. Organizations must not only maintain accurate and reliable data but also ensure that sensitive information is properly managed, protected, and anonymized. Non-compliance with these regulations not only carries financial penalties but also damages an organization's reputation. As a result, data quality assurance strategies need to account for data privacy considerations, leading to the development of tools and processes that safeguard data while maintaining its quality.
In the realm of data engineering, ensuring reliable and trustworthy data is paramount. Data Quality Assurance plays a pivotal role in this pursuit, acting as the foundation for informed decision-making and successful data-driven initiatives. By implementing rigorous data validation, cleansing, and monitoring processes, organizations can mitigate errors, enhance data accuracy, and foster a culture of data integrity. Ultimately, prioritizing Data Quality Assurance safeguards against misinformation and empowers businesses to harness the full potential of their data assets, driving innovation and growth.