Data Quality: The Foundation of Effective Business Analytics
Discover the significance of data quality in business analytics. Explore key components, best practices, and real-world examples, and learn how to ensure high-quality data for informed decision-making.
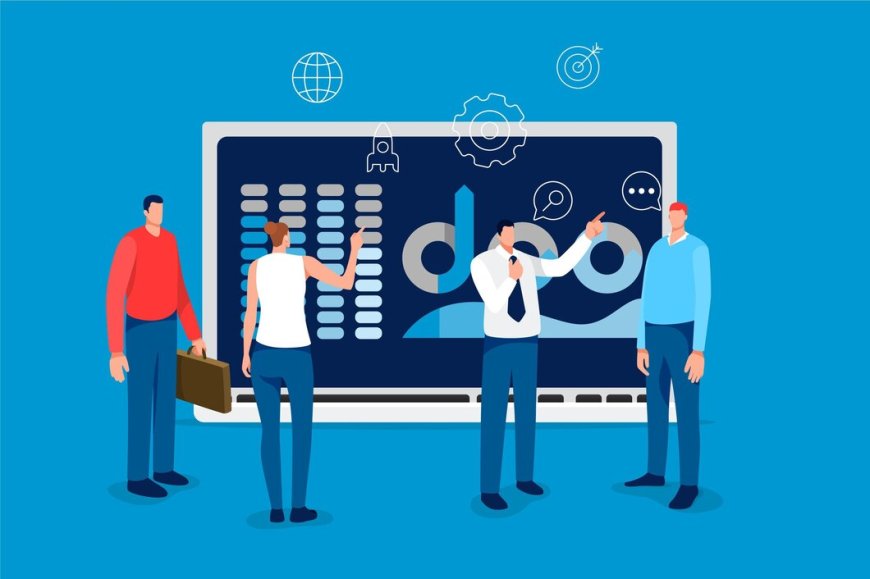
Data Quality is the cornerstone upon which effective business analytics stands. In today's data-driven world, organizations rely heavily on data analytics to make informed decisions, gain insights, and stay competitive. However, the value of analytics is directly dependent on the quality of the data used. Poor data quality can lead to erroneous conclusions, misguided strategies, and financial losses.
Understanding Data Quality
Understanding data quality is essential for any organization seeking to harness the power of business analytics. Data quality refers to the reliability and accuracy of data, and it encompasses various aspects such as accuracy, completeness, consistency, timeliness, and relevance. Each of these components plays a crucial role in ensuring that the data used for analytics is not only abundant but also trustworthy.
Accuracy involves the correctness of data, ensuring that the information reflects the real-world scenario it is intended to represent. Completeness refers to having all the necessary data points, without missing or omitted values, to provide a holistic view. Consistency ensures that data is uniform across various sources and does not present conflicting information. Timeliness emphasizes the importance of having data available when needed, without significant delays, to support timely decision-making. Relevance ensures that the data being collected and analyzed aligns with the specific needs and objectives of the analytics process.
Common data quality issues, such as data entry errors, duplicate data, missing values, inconsistent formats, and data decay, can significantly compromise the quality of analytics outcomes. Organizations must be vigilant in addressing these issues to maintain data integrity and reliability.
In understanding data quality, organizations often employ data profiling and analysis techniques to assess the condition of their data. This involves examining the structure, content, and relationships within datasets to identify anomalies and inconsistencies. By understanding the strengths and weaknesses of their data, organizations can take proactive measures to enhance data quality.
The Impact of Poor Data Quality
The impact of poor data quality on organizations is profound and multifaceted, influencing various aspects of business operations and decision-making. One of the primary consequences is inaccurate decision-making. When organizations rely on flawed or incomplete data, the decisions derived from such information are inherently compromised. This can lead to misguided strategies, ineffective resource allocation, and missed opportunities. The integrity of business intelligence and analytics is contingent on the quality of the underlying data, and any deficiencies in this regard can have a cascading effect throughout an organization.
Moreover, poor data quality erodes trust. Stakeholders, including customers, investors, and internal teams, depend on accurate and reliable information to make informed decisions. When data quality is compromised, trust in the organization's competence diminishes. This loss of confidence can manifest in customer dissatisfaction, increased skepticism from investors, and a decline in employee morale. Trust is a fragile asset, and once compromised, it is challenging to rebuild.
Financial implications are another critical facet of the impact of poor data quality. Organizations may incur significant costs in rectifying errors, conducting damage control, and dealing with legal consequences if data inaccuracies lead to regulatory non-compliance. Additionally, poor data quality can result in missed business opportunities and revenue losses. For instance, marketing efforts based on inaccurate customer data may fail to reach the target audience effectively, leading to suboptimal sales outcomes.
Data Quality Standards and Best Practices
In the world of data analytics, maintaining high data quality is essential to ensure that insights and decisions drawn from data are accurate and reliable. Data quality standards and best practices are a set of guidelines, frameworks, and procedures designed to help organizations achieve and maintain data of the highest quality. These standards and practices are crucial for ensuring that data is accurate, complete, consistent, and timely and that it meets the specific needs of the organization. Here are some key points to consider
Data Quality Frameworks: There are various data quality frameworks available, such as ISO 8000, Six Sigma, and Total Data Quality Management (TDQM). These frameworks provide a structured approach to evaluating, improving, and maintaining data quality within an organization. ISO 8000, for example, offers a comprehensive set of standards for data quality, covering aspects like accuracy, completeness, and consistency.
Data Governance: Effective data governance is fundamental to maintaining data quality. Data governance involves the establishment of clear roles and responsibilities, data stewardship, and the creation of data quality policies and procedures. By implementing data governance practices, organizations can ensure that data is managed, protected, and utilized consistently across the enterprise.
Data Quality Tools and Technologies: A wide range of tools and technologies are available to assist organizations in managing and enhancing data quality. Data cleaning software, for instance, can help identify and rectify data entry errors, remove duplicates, and address missing values. Master Data Management (MDM) systems are another technology used to create a single, authoritative source of truth for critical data elements.
Data Quality Auditing: Regular data quality audits are essential for monitoring and maintaining the integrity of data. These audits involve examining data to identify anomalies, inconsistencies, and errors. They also help in assessing the effectiveness of data quality processes and identifying areas for improvement.
Ensuring Data Quality in Business Analytics
Ensuring Data Quality in Business Analytics is a crucial process that involves various steps and practices to guarantee that the data used for analytical purposes is accurate, reliable, and consistent. Without high data quality, the insights and decisions derived from business analytics can be flawed, leading to costly mistakes and poor business outcomes. Here's an explanation of this topic:
Data Preparation and Cleaning: Data quality assurance begins with the process of data preparation and cleaning. This involves identifying and addressing issues such as missing data, data entry errors, duplicate records, and inconsistent data formats. Cleaning data ensures that the information you're working with is accurate and complete.
Data Profiling and Analysis: Data profiling involves assessing the overall quality of the dataset. Analysts use various statistical and data profiling techniques to identify anomalies, outliers, and patterns within the data. By understanding the data's characteristics, you can uncover potential issues and areas that require improvement.
Data Validation and Verification: Data validation and verification processes check data against predefined rules and standards. For instance, validation checks can ensure that numerical data falls within acceptable ranges, or that text data adheres to specific formats. Verification involves confirming that data is correct by cross-referencing it with external sources or conducting additional research.
Data Quality Assurance Processes: Establishing data quality assurance processes is essential. This includes defining responsibilities for data quality, setting up workflows for data review and validation, and implementing procedures for handling data discrepancies. Data quality assurance may also involve implementing data quality rules and triggers to alert stakeholders to potential issues.
Benefits of High Data Quality
High data quality is a cornerstone of effective business analytics, offering a myriad of benefits that directly impact an organization's overall performance and success. One of the primary advantages is the substantial improvement in decision-making processes. Accurate and reliable data ensures that executives and decision-makers have a trustworthy foundation upon which to base their strategic choices. Informed decisions lead to better outcomes, greater operational efficiency, and a competitive edge in the marketplace.
Beyond decision-making, high data quality contributes to enhanced customer satisfaction. Inaccurate or inconsistent data can lead to mistakes in customer interactions, eroding trust and satisfaction. With reliable data, organizations can provide personalized and targeted services, building stronger relationships with customers and fostering loyalty.
In a competitive business landscape, maintaining high data quality provides a distinct advantage. Organizations with clean and reliable data can respond more quickly to market changes, identify emerging trends, and adapt their strategies accordingly. This agility is critical in an environment where rapid innovation and responsiveness to customer needs are paramount.
Moreover, adherence to data quality standards contributes to regulatory compliance. Many industries are subject to strict data protection and privacy regulations. Ensuring the accuracy and security of data not only helps in compliance but also protects the organization from legal and financial repercussions.
Challenges in Maintaining Data Quality
Big Data and Data Volume: One of the primary challenges in maintaining data quality is dealing with the sheer volume of data generated by businesses today. With the advent of Big Data, organizations are accumulating massive amounts of information at an unprecedented rate. This data deluge poses challenges in terms of processing, storing, and, most importantly, ensuring data quality. As the volume of data increases, it becomes increasingly difficult to identify and rectify errors, leading to the potential for data degradation and loss of accuracy.
Data Privacy and Security Concerns: Data quality is not just about accuracy; it also encompasses the security and privacy of data. As organizations collect and process sensitive information, maintaining data quality while adhering to stringent data protection regulations can be challenging. Ensuring that data is accurate, consistent, and complete while safeguarding it from unauthorized access, breaches, and cyber threats adds a layer of complexity to data quality management. Balancing the need for data quality with stringent privacy and security requirements is a delicate task.
Scalability and Automation: Achieving and maintaining data quality can be relatively straightforward in small-scale projects. However, as businesses grow and expand, managing data quality becomes increasingly complex. Maintaining consistency, accuracy, and timeliness across a growing volume of data requires scalable data quality processes and automation. Implementing these systems often involves significant investment in technology and expertise, and organizations must adapt to these evolving needs.
Future Trends in Data Quality
The landscape of data quality is continually evolving, and as technology advances, new trends emerge that shape the future of managing and ensuring data quality. One prominent trend is the integration of artificial intelligence (AI) and machine learning (ML) into data quality processes. AI and ML algorithms can be employed to automate the detection and correction of data errors, anomalies, and inconsistencies. These technologies have the potential to significantly enhance the efficiency of data quality management by allowing systems to learn from patterns and continuously improve accuracy.
Another noteworthy trend is the exploration of blockchain technology for maintaining data integrity. Blockchain, which is well-known for its application in secure and transparent transactions, is being considered as a means to establish and verify the authenticity of data. By creating an immutable and decentralized ledger, blockchain can prevent unauthorized alterations to data, ensuring a higher level of trust and security in the information being used for analytics.
Furthermore, the future of data quality will likely witness the evolution of standards and regulations. As the importance of data quality becomes more widely recognized, governments and industry bodies may introduce new guidelines and standards to govern how organizations manage, store, and process data. Compliance with these evolving standards will be crucial for businesses to maintain a competitive edge, build trust among stakeholders, and mitigate risks associated with data-related issues.
Data quality is undeniably the cornerstone of effective business analytics. It ensures that the insights drawn from data are accurate, reliable, and actionable, ultimately influencing sound decision-making and driving business success. Neglecting data quality can lead to costly errors and erode trust in the analytics process. By adhering to data quality standards, best practices, and emerging technologies, organizations can harness the full potential of their data, gain a competitive edge, and pave the way for a data-driven future in business analytics.