Machine Learning in Finance: Revolutionizing the Financial Industry
Discover how data-driven algorithms, predictive modeling, and AI-powered insights are reshaping investment strategies and risk management
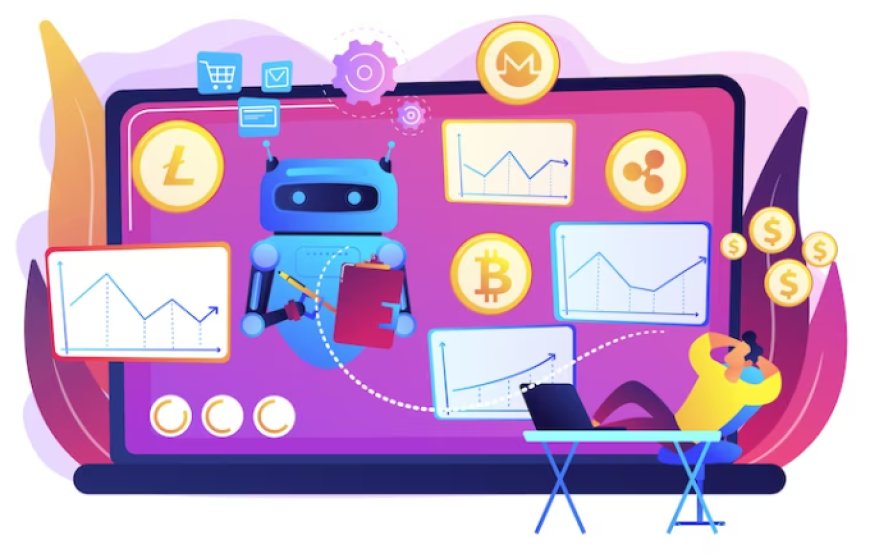
Machine learning is revolutionizing the financial industry, reshaping traditional practices and driving unprecedented advancements. With its ability to process vast amounts of data, machine learning enhances efficiency, risk management, and customer experience in finance. This transformative technology has opened new possibilities for fraud detection, credit risk assessment, algorithmic trading, and personalized services. As the financial landscape continues to evolve, machine learning stands as a powerful tool, propelling the industry into a new era of innovation and growth.
Machine Learning
Machine learning is a subset of artificial intelligence that empowers computers to learn and improve from experience without explicit programming. It involves algorithms that analyze data, identify patterns, and make predictions or decisions based on the patterns detected. Machine learning has diverse applications across various industries, enabling automation, data-driven insights, and the development of intelligent systems.
Importance of machine learning in finance
-
Enhanced Fraud Detection: Machine learning algorithms can identify fraudulent activities and transactions in real-time, minimizing financial losses and protecting customers.
-
Improved Credit Risk Assessment: Predictive modeling enables more accurate credit scoring, allowing lenders to assess default probabilities and make better lending decisions.
-
Efficient Algorithmic Trading: Automated trading strategies based on machine learning algorithms analyze market data, enabling faster and more informed trading decisions.
-
Personalized Customer Experience: Machine learning enables personalized recommendations, offers, and services tailored to individual customers' needs and preferences.
-
Efficient Data Analysis: Machine learning algorithms process vast amounts of financial data quickly, enabling faster and more data-driven decision-making.
-
Risk Management: Machine learning helps financial institutions identify and manage various risks, including credit risk, market risk, and operational risk.
-
Portfolio Management: Machine learning models can optimize investment portfolios, balancing risk and return to achieve better outcomes for investors.
-
Customer Segmentation: Machine learning helps in segmenting customers based on their behavior, allowing targeted marketing and customer retention strategies.
-
Compliance and Regulatory Reporting: Machine learning assists financial institutions in meeting compliance requirements and streamlining regulatory reporting processes.
-
Predictive Analytics: Machine learning enables the prediction of market trends, customer behavior, and other financial variables, aiding in strategic planning and decision-making.
Machine Learning Applications in Finance
Machine learning applications in finance are diverse and impactful, transforming how financial institutions operate and serve their customers. Some key applications include:
-
Fraud Detection and Security : Machine learning algorithms analyze transactions in real-time, detecting unusual patterns and flagging potential fraudulent activities instantly. Machine learning can identify complex fraud patterns that may go unnoticed by traditional rule-based systems, enhancing fraud detection accuracy.
-
Credit Risk Assessment : Machine learning models assess an individual's creditworthiness by analyzing various data points, leading to more accurate credit scoring and lending decisions.Machine learning algorithms can predict the probability of loan defaults, enabling lenders to manage credit risk more effectively.
-
Algorithmic Trading: Machine learning powers automated trading systems that execute trades based on predefined algorithms, optimizing trading strategies for efficiency and profitability. Machine learning models analyze historical market data and trends to predict future price movements, assisting traders in making informed decisions.
-
Customer Relationship Management: Machine learning algorithms analyze customer behavior and preferences to offer personalized product recommendations and targeted marketing campaigns.Machine learning can predict customer churn, allowing financial institutions to implement retention strategies and improve customer loyalty.
These machine learning applications have become indispensable in the financial industry, providing actionable insights, improving efficiency, and driving better customer experiences. As the technology continues to evolve, its role in finance is expected to expand, further revolutionizing the way financial services are delivered and consumed.
Advantages and Benefits of Machine Learning in Finance
Machine learning has brought a wave of transformation to the financial industry, offering a multitude of advantages that enhance efficiency, risk management, and customer experiences.
-
Improved Efficiency: Machine learning automates manual processes, liberating financial professionals from repetitive tasks. This automation not only saves time but also reduces the risk of human errors, leading to more accurate and reliable results. Moreover, the speed and agility of machine learning algorithms enable faster decision-making and data analysis. Financial institutions can process vast amounts of data in real-time, gaining valuable insights to respond swiftly to market changes and emerging trends.
-
Enhanced Risk Management: Machine learning empowers financial institutions with sophisticated risk assessment tools. By analyzing large and diverse datasets, machine learning algorithms can identify potential risks and vulnerabilities, enabling better risk mitigation strategies. Furthermore, machine learning's ability to detect patterns and anomalies in real-time enhances fraud detection. Financial institutions can proactively identify suspicious activities and prevent fraudulent transactions, safeguarding both their assets and their customers' trust.
-
Better Customer Experience : Personalization has become a key driver of customer satisfaction, and machine learning plays a crucial role in delivering tailored experiences. By analyzing customer behavior and preferences, financial institutions can offer personalized services and customized product recommendations. This level of personalization strengthens customer loyalty and engagement. Additionally, machine learning streamlines customer interactions through chatbots and virtual assistants, providing seamless and efficient customer support.
Machine learning's advantages in finance have revolutionized the industry. It optimizes processes, improves risk management, and elevates customer experiences, positioning financial institutions to thrive in the era of digital disruption. Embracing machine learning, the financial sector can drive innovation, achieve operational excellence, and stay ahead in a competitive landscape.
Challenges and Limitations of Machine Learning in Finance
While machine learning offers significant benefits to the financial industry, it also presents various challenges and limitations that must be carefully addressed:
Data Privacy and Security Concerns: One of the most critical challenges in using machine learning in finance is data privacy and security. Financial institutions deal with sensitive customer information, and any data breaches or mishandling can have severe consequences. Ensuring robust data privacy measures, encryption, and secure data storage are essential to protect customer data and maintain trust.
Model Interpretability and Explainability: Machine learning models, particularly complex deep learning algorithms, often lack transparency in their decision-making process. This lack of interpretability can be a concern in highly regulated industries like finance, where explainability is crucial for compliance and audit purposes. Interpretable AI methods are needed to understand how models arrive at specific predictions, particularly in critical decisions like credit approvals.
Regulatory Compliance and Transparency: The financial industry operates under strict regulatory frameworks designed to protect consumers and maintain market integrity. Integrating machine learning into financial processes requires compliance with regulations such as Basel III, GDPR, and Anti-Money Laundering (AML) guidelines. Meeting these requirements can be challenging due to the complexity of machine learning models and the need to demonstrate transparency in their functioning.
Data Quality and Bias: Machine learning algorithms heavily rely on training data to make accurate predictions. Ensuring the quality, accuracy, and representativeness of the data used for training is vital to avoid biased results. Biases in data can lead to discriminatory outcomes, impacting credit decisions, loan approvals, and customer experiences.
Overfitting and Generalization: Machine learning models may sometimes overfit to the training data, meaning they perform well on the training set but fail to generalize to new, unseen data. Overfitting can lead to unreliable predictions and inaccurate risk assessments, undermining the effectiveness of the models in real-world scenarios.
Future Trends and Opportunities in Machine Learning for Finance
As technology continues to evolve, machine learning in finance is expected to witness significant advancements and open up new opportunities. Some of the key future trends are:
Advancements in Deep Learning and NLP for Finance
-
Deep learning, a subset of machine learning, holds immense potential for finance. Advancements in deep neural networks will enable more accurate predictions and better handling of complex financial data, such as time series and unstructured data.
-
NLP will play a crucial role in sentiment analysis, extracting insights from news articles, social media, and financial reports. Improved NLP models will enhance automated trading strategies and decision-making processes.
Integration of AI and Blockchain in Financial Services
-
The integration of AI and blockchain technology will facilitate the development of smart contracts. AI-powered smart contracts can automatically execute financial agreements based on predefined conditions, improving efficiency and reducing human intervention.
-
AI can be combined with blockchain to enhance fraud prevention. Immutable blockchain records can serve as reliable data sources for AI algorithms, improving fraud detection and security in financial transactions.
-
AI-powered algorithms can help optimize DeFi platforms, providing personalized financial services and automating various DeFi protocols, such as lending, trading, and asset management.
Continued Growth of Fintech Startups leveraging Machine Learning
-
Fintech startups will leverage machine learning to offer personalized financial products and services, catering to the unique needs and preferences of customers.
-
Machine learning algorithms will enable fintech startups to develop alternative credit scoring models, expanding access to credit for underserved populations.
-
Fintech startups will continue to innovate in fraud detection, leveraging machine learning to build robust systems that protect customers and businesses from financial fraud.
Machine learning is revolutionizing the financial industry, offering numerous advantages such as improved efficiency, enhanced risk management, and better customer experiences. However, as financial institutions adopt AI-driven solutions, addressing ethical considerations becomes crucial. Prioritizing data privacy, fairness, transparency, and accountability will ensure responsible AI deployment, fostering trust and creating a more sustainable and inclusive financial landscape. By embracing ethical AI practices, the financial industry can harness the full potential of machine learning while upholding its commitment to ethical decision-making and customer well-being.