Measuring Environmental Impact with Business Analytics
Explore the transformative power of business analytics in measuring and mitigating environmental impact. Learn how data-driven insights are shaping sustainable business practices for a greener future.
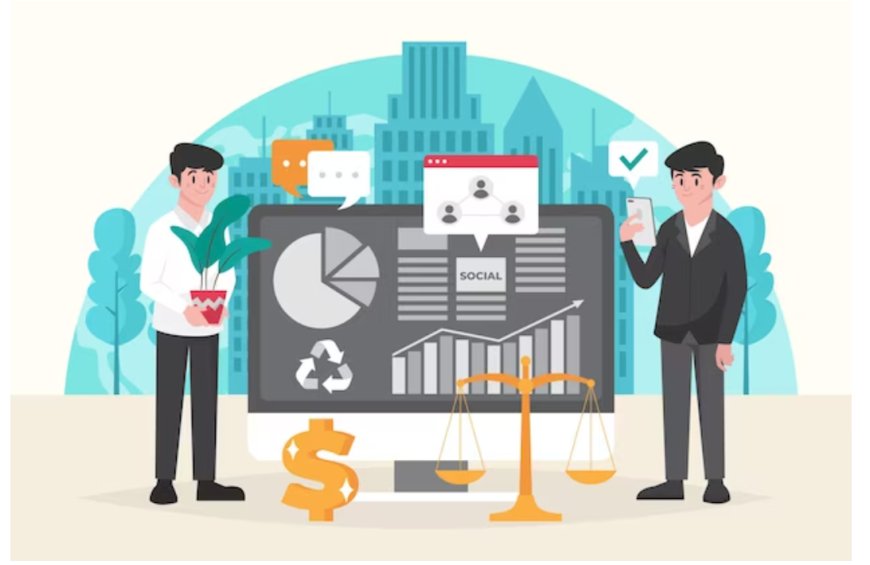
In a world where corporate responsibility is no longer just a buzzword but a crucial aspect of business success, the integration of business analytics has emerged as a transformative force in the pursuit of environmental sustainability. This paradigm shift recognizes the imperative for organizations to not only quantify their environmental impact but to do so with precision, insight, and strategic intent. Measuring environmental impact with business analytics involves harnessing the power of data to understand, optimize, and ultimately reduce the ecological footprint of businesses.
Understanding Environmental Impact
In comprehending the environmental impact of businesses, it is essential to delve into the various dimensions that contribute to their ecological footprint. One of the primary facets is carbon emissions, which arise from activities such as energy consumption, transportation, and production processes. Businesses emit greenhouse gases, chiefly carbon dioxide, which contribute to climate change. Measuring and managing carbon emissions is a crucial component of any sustainability strategy.
Another critical dimension is water usage, a resource that is increasingly scarce in many parts of the world. Businesses draw upon water for various processes, including manufacturing and cooling. Understanding and quantifying water usage is vital for ensuring responsible and sustainable resource management.
Waste generation constitutes the third dimension of environmental impact. Businesses produce a myriad of waste types, including solid waste, wastewater, and electronic waste. Improper disposal or management of these wastes can lead to pollution and harm ecosystems. Thus, businesses need to measure and reduce waste generation to minimize their negative impact on the environment.
In the past, businesses relied on traditional methods for assessing their environmental impact. These methods often involved periodic audits and manual assessments, providing only a snapshot of the environmental performance. This approach lacked the ability to offer real-time insights or a comprehensive understanding of the complex interplay between various activities and their environmental consequences.
Optimizing Resource Utilization
Optimizing resource utilization is a critical aspect of sustainable business practices, and it involves the strategic management of resources to ensure maximum efficiency and minimal waste. In the context of environmental sustainability, businesses are increasingly turning to data-driven approaches, specifically through business analytics, to analyze and optimize their resource consumption. This process involves collecting and analyzing data related to energy usage, water consumption, raw materials, and other inputs across various operational facets. By employing analytics tools, organizations gain insights into patterns, trends, and inefficiencies in their resource utilization.
Business analytics enables companies to identify areas where resources are underutilized or wasted, facilitating the development of targeted strategies for improvement. For instance, energy consumption patterns can be scrutinized to pinpoint times of excessive use or areas where energy-efficient technologies can be implemented. Water usage data can reveal opportunities for conservation and recycling. Additionally, the analysis of raw material inputs can lead to more sustainable sourcing practices and reductions in overall consumption.
This approach not only benefits the environment but also has significant economic implications. Businesses can realize cost savings by optimizing resource utilization, as efficiency improvements often translate into reduced operational expenses. Moreover, it aligns with the broader goals of corporate social responsibility, demonstrating a commitment to sustainable practices that resonate positively with customers, investors, and other stakeholders.
Challenges in Implementing Business Analytics for Environmental Impact
Data Quality
-
Explanation: The accuracy and reliability of analytics heavily depend on the quality of the data used. In the context of environmental impact measurement, ensuring that the data collected is accurate, consistent, and representative of the actual processes is a common challenge. Inaccurate or incomplete data can lead to flawed insights, hindering the effectiveness of sustainability strategies.
-
Example: If emission data is not accurately recorded or if there are gaps in the data, it may lead to underestimation or overestimation of the environmental impact, impacting decision-making.
System Integration
-
Explanation: Many organizations operate on complex and diverse systems for different business functions. Integrating these systems to collect, process, and analyze environmental impact data can be challenging. Incompatibility between existing systems and analytics tools may result in data silos, making it difficult to gain a comprehensive view of the organization's environmental performance.
-
Example: The data collected from energy management systems may need to be integrated with supply chain data to provide a holistic view of the environmental impact, but the integration process may encounter technical obstacles.
Skilled Workforce
-
Explanation: Implementing and managing business analytics for environmental impact requires a workforce with a specific skill set. Data scientists, environmental analysts, and sustainability experts are needed to interpret data, create models, and derive meaningful insights. A shortage of skilled professionals in these areas can impede the successful integration of analytics into sustainability strategies.
-
Example: Organizations may struggle to find professionals who understand both the intricacies of environmental science and the complexities of data analytics, making it challenging to derive actionable insights from the data.
The Promise of AI and ML in Enhancing Analytics for Sustainability
Advanced Predictive Modeling
-
Explanation: Artificial Intelligence (AI) and Machine Learning (ML) can enhance predictive modeling capabilities. These technologies can analyze historical data to identify patterns and trends, allowing organizations to predict future environmental impacts with greater accuracy. This forward-looking approach enables proactive decision-making and risk mitigation.
-
Example: AI algorithms can analyze past energy consumption patterns and predict future demand, helping businesses optimize energy usage and reduce their carbon footprint.
Real-time Monitoring and Response
-
Explanation: AI and ML enable real-time monitoring of environmental parameters. This capability allows organizations to respond promptly to changes in environmental performance, reducing the likelihood of negative impacts. Real-time insights empower businesses to make swift adjustments to their operations for maximum sustainability.
-
Example: ML algorithms can continuously monitor air and water quality, providing instant alerts if certain thresholds are exceeded, enabling rapid intervention to prevent environmental harm.
Automated Decision-Making
-
Explanation: AI can automate decision-making processes based on predefined sustainability criteria. This reduces the burden on human resources and ensures that decisions align with environmental goals. Automated decision-making also facilitates quicker responses to dynamic environmental challenges.
-
Example: An AI system can automatically adjust production schedules based on energy consumption forecasts and emission reduction targets, optimizing operations for sustainability.
The integration of business analytics into environmental sustainability strategies is a pivotal step towards responsible and successful business operations. By quantifying impact, identifying hotspots, optimizing resource utilization, and leveraging predictive modeling, organizations can navigate the complex landscape of environmental responsibility more effectively. While challenges persist, such as data quality and integration, the promise of AI and ML technology holds the key to even more sophisticated assessments. Embracing these tools is not only a choice but a strategic imperative for businesses looking to thrive in an environmentally conscious world. In the quest for a sustainable future, business analytics is a compass guiding us towards greener, more responsible, and ultimately more successful endeavors.