The Future of Data Analytics: AI and Machine Learning Trends
Explore the transformative potential of AI and Machine Learning in the future of data analytics. Discover emerging trends and challenges in this evolving landscape.
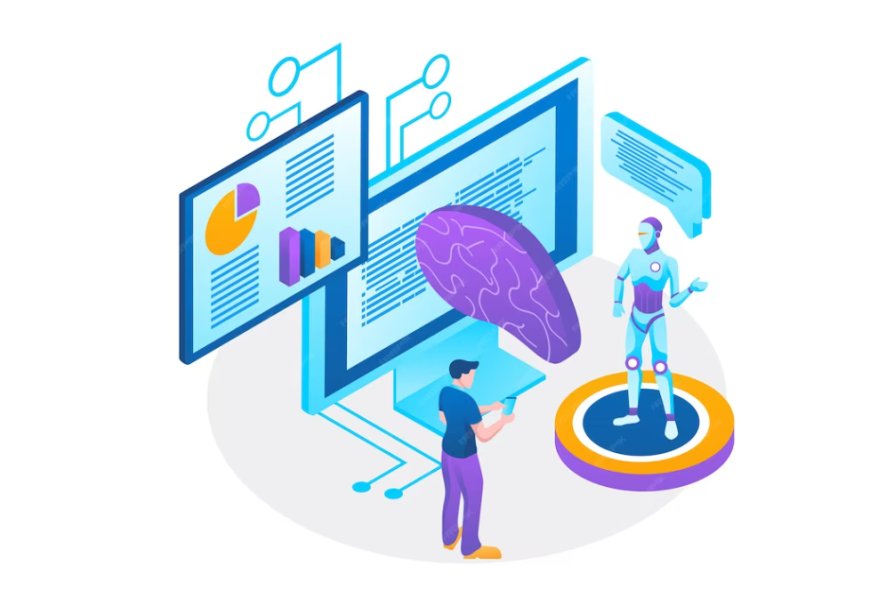
The future of data analytics is on the brink of a transformative revolution, driven by the rapid advancement of Artificial Intelligence (AI) and Machine Learning (ML) technologies. In this era of big data, businesses, industries, and researchers are harnessing the power of AI and ML to unlock unprecedented insights from vast datasets. This fusion of cutting-edge technologies promises to reshape the way we analyze, interpret, and utilize data in the coming years.
Historical Overview of Data Analytics
The historical overview of data analytics provides valuable insights into the evolution of techniques and methodologies used to analyze data throughout human history. From rudimentary methods employed in ancient civilizations to the sophisticated tools and technologies of the modern era, this historical context helps us appreciate the significant advancements that have shaped the field of data analytics.
In the early stages of human civilization, data analysis was rudimentary, relying on basic arithmetic and manual calculations to derive insights from limited datasets. Ancient civilizations, such as the Babylonians and Egyptians, used data analysis techniques for purposes like tracking agricultural yields, predicting astronomical events, and managing resources.
The Industrial Revolution marked a turning point in data analysis, with the introduction of mechanized data processing tools like punch cards and mechanical calculators. These innovations significantly improved the speed and accuracy of data manipulation, enabling the emergence of early statistical techniques.
The mid-20th century saw the rise of modern statistics and the development of electronic computers, which revolutionized data analysis. Pioneers like John Tukey and Norbert Wiener laid the foundation for statistical methods, while the advent of computers made it possible to process and analyze larger datasets more efficiently. This era also saw the emergence of business intelligence tools for decision support.
With the growth of the internet and the digital age, data analytics expanded exponentially. The 21st century witnessed the rise of big data, where organizations began collecting vast amounts of data from various sources. This led to the development of data warehousing, data mining, and predictive analytics techniques, enabling businesses to gain deeper insights into customer behavior and trends.
Current State of Data Analytics
The current state of data analytics is characterized by an unprecedented surge in its significance across various industries and domains. Data analytics has evolved from being a mere business tool to becoming a cornerstone of decision-making processes in today's interconnected world. Major players in the technology industry, including Google, Amazon, and Microsoft, have invested heavily in developing advanced analytics platforms, making data analytics more accessible and powerful than ever before.
In the business realm, organizations of all sizes and sectors are increasingly relying on data analytics to gain a competitive edge. From e-commerce companies optimizing customer experiences through recommendation algorithms to healthcare institutions using predictive analytics for patient care, the applications of data analytics are vast and diverse.
The current state of data analytics is also marked by the convergence of data sources. The proliferation of the Internet of Things (IoT) devices, social media platforms, and sensors has led to an explosion in data generation. This wealth of data, often referred to as big data, has necessitated the use of advanced analytics techniques, including AI and machine learning, to extract meaningful insights.
AI and Machine Learning in Data Analytics
AI (Artificial Intelligence) and Machine Learning (ML) are revolutionizing the field of data analytics by introducing automation, predictive capabilities, and advanced pattern recognition. Here's a more detailed explanation of their role in data analytics:
-
Automation of Data Analysis: AI and ML algorithms can automatically process and analyze massive datasets at incredible speeds. They can handle repetitive tasks such as data cleaning, transformation, and basic analysis without human intervention.
-
Predictive Analytics: One of the most significant contributions of AI and ML to data analytics is predictive modeling. Machine learning models can learn from historical data to make predictions about future events or outcomes. For instance, businesses can use predictive analytics to forecast sales, detect anomalies in financial transactions, or predict equipment failures in manufacturing.
-
Pattern Recognition: ML algorithms excel at identifying complex patterns and trends in data that might be too subtle or numerous for humans to notice. This is especially valuable for customer behavior analysis, fraud detection, and anomaly detection in various domains.
-
Personalization: AI-powered recommendation systems are used extensively in e-commerce, entertainment, and content platforms. These systems analyze user behavior and preferences to provide personalized product recommendations, content suggestions, or advertising, enhancing user engagement and satisfaction.
-
Natural Language Processing (NLP): NLP is a subset of AI that focuses on understanding and processing human language. It is crucial in sentiment analysis, chatbots, and text mining for extracting valuable insights from unstructured text data, such as customer reviews, social media posts, and news articles.
Emerging Trends in Data Analytics
Emerging trends in data analytics are shaping the future of how organizations leverage data for informed decision-making and strategy development. These trends represent a response to the growing complexity and volume of data available in the digital age, and they encompass various aspects of data analytics, from technology to ethics.
One prominent trend is the emphasis on explainability and transparency in AI models. As AI and machine learning models become increasingly sophisticated, there is a growing need to understand how these models arrive at their decisions. Explainability is crucial not only for compliance and ethical reasons but also for building trust in AI systems. Organizations are investing in research and tools that make AI more interpretable, ensuring that the decision-making processes are more accessible and comprehensible to humans.
Another significant trend is the adoption of edge computing and real-time analytics. With the proliferation of Internet of Things (IoT) devices and the need for instant insights, data analytics is moving closer to the data source. Edge computing allows organizations to process data at or near the data source, reducing latency and enabling real-time decision-making. This trend is especially vital in fields like autonomous vehicles, healthcare, and manufacturing, where split-second decisions can have a profound impact.
Future Applications of Data Analytics
"Future Applications of Data Analytics" refers to the various domains and industries where data analytics, powered by AI and machine learning, is expected to make significant advancements and have a profound impact in the years to come. These applications represent cutting-edge uses of data analytics techniques and technologies to solve complex problems, make informed decisions, and drive innovation. Here are some key areas where future applications of data analytics are anticipated:
-
Healthcare and Predictive Medicine: Data analytics can revolutionize healthcare by analyzing patient data to predict diseases, optimize treatment plans, and enhance patient outcomes. AI-driven diagnostics, personalized medicine, and real-time health monitoring are some of the future applications.
-
Financial Services and Risk Management: In the financial sector, data analytics is used to detect fraudulent transactions, assess credit risks, and optimize investment portfolios. Future applications may include more sophisticated fraud detection algorithms, automated financial advising, and real-time market predictions.
-
Manufacturing and Supply Chain Optimization: Data analytics can optimize production processes, reduce downtime, and enhance supply chain efficiency. Future applications include predictive maintenance, demand forecasting, and autonomous production systems.
-
Autonomous Vehicles and Transportation: Self-driving cars and smart transportation systems rely on data analytics for real-time decision-making. Future applications may involve improved route planning, traffic management, and safety enhancements.
Challenges and Concerns
As we navigate the evolving landscape of data analytics propelled by AI and Machine Learning, a host of challenges and concerns emerge that demand careful consideration. One pressing issue is the paramount importance of data security and privacy. With the increasing volume and sensitivity of data being analyzed, safeguarding against unauthorized access and data breaches becomes a critical priority. Additionally, the inherent risk of bias in AI models raises ethical concerns, as algorithms may inadvertently perpetuate and amplify existing societal prejudices.
Data governance and compliance pose significant challenges, particularly in navigating the complex regulatory frameworks surrounding data usage and storage. Another hurdle is the widening skill gap in AI and Machine Learning, as the demand for professionals proficient in these technologies outpaces the current supply. Striking a balance between innovation and responsible use is essential to address these challenges and ensure that the future of data analytics remains ethical, secure, and inclusive. Overcoming these obstacles will be pivotal in realizing the full potential of AI and Machine Learning in the realm of data analytics.
Potential Impact on Society
The potential impact of the evolving landscape of data analytics, driven by AI and Machine Learning trends, extends far beyond the realm of business and technology, reaching deep into the fabric of society. This transformative force is poised to bring about significant changes that will ripple across various domains.
Economically, the integration of AI and ML into data analytics is expected to fuel innovation and productivity, potentially leading to economic growth and job creation. However, it may also disrupt traditional employment patterns, requiring a rethinking of workforce skills and job roles. Businesses that harness these technologies effectively are likely to gain a competitive edge, while those that do not risk falling behind.
On a social level, the impact is profound. AI-powered analytics can help solve complex societal issues, such as healthcare diagnostics and treatment optimization, urban planning, and disaster response. Additionally, these technologies can enhance accessibility, convenience, and personalization across various services, from education to entertainment. However, concerns about privacy, algorithmic bias, and ethical considerations must be addressed to ensure that these advances benefit society as a whole and do not exacerbate existing inequalities.
The future of data analytics, propelled by the relentless march of AI and Machine Learning, holds immense promise for innovation and insight generation. As we navigate this dynamic landscape, the integration of these technologies is not merely a trend but a fundamental shift in how we approach data. The potential applications across diverse sectors, from healthcare to finance and beyond, underscore the transformative impact that lies ahead. However, as we embrace this future, it is essential to remain vigilant about ethical considerations, data privacy, and the equitable deployment of these technologies. The journey into the future of data analytics is one of continuous evolution, and as we adapt to these advancements, it is crucial to foster a responsible and inclusive approach that ensures the benefits are shared by all.