The Rise of Ethical Data Science: the Moral Landscape
Explore the ethical dimensions of data science in 'The Rise of Ethical Data Science: the Moral Landscape.' Discover how data-driven decisions impact society. Dive into the world of responsible data science.
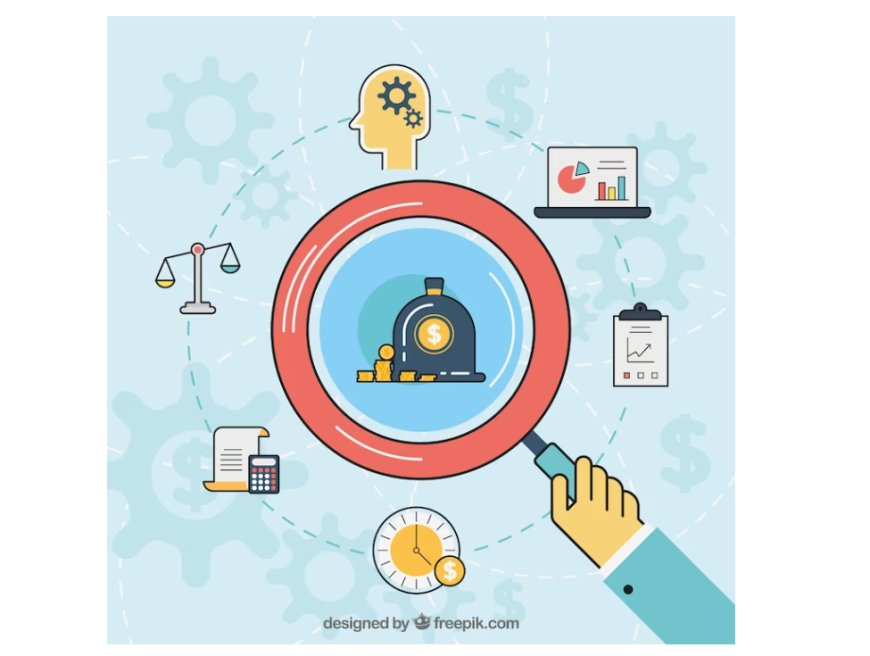
In the era of big data, where information flows like a torrential river, the role of data science has become pivotal in shaping our world. However, with great power comes great responsibility, and the ethical implications of data science practices have come under intense scrutiny. The rise of ethical data science marks a paradigm shift, emphasizing the moral landscape that underlies the collection, analysis, and application of data.
Foundations of Ethical Data Science
Ethical data science serves as the moral compass for the rapidly evolving field of data science, guiding practitioners and organizations to navigate the complex landscape of data analytics and technology in an ethically responsible manner. At its core, ethical data science is founded upon a set of principles and values that emphasize fairness, transparency, accountability, and respect for individual rights. These foundational principles provide the groundwork for ethical decision-making throughout the entire data science lifecycle.
One of the fundamental aspects of ethical data science is defining what it means to be ethical in this context. It involves recognizing that data science, by its very nature, wields immense power over individuals and society as a whole. The process of collecting, analyzing, and interpreting data can significantly impact people's lives, from shaping public policies to influencing personal decisions. Consequently, ethical data science strives to ensure that this power is used responsibly and that the benefits of data science are distributed equitably.
Historically, ethical considerations have always been present in various forms within scientific research and technological advancements. However, the rise of data science and the proliferation of data-driven technologies have brought these ethical concerns into sharper focus. The scale and speed at which data is collected and processed, coupled with the increasing complexity of algorithms and machine learning models, have raised new ethical challenges. This necessitates a dedicated and structured approach to ethics within data science.
The Need for Ethical Data Science
The Need for Ethical Data Science stems from the increasing recognition of the potential risks and consequences associated with the vast and often unchecked use of data. In a digital era where data has become a cornerstone of innovation, decision-making, and technological advancements, ethical considerations are paramount.
One critical aspect is the preservation of privacy, as individuals' personal information is frequently collected, analyzed, and utilized for various purposes. The mishandling of sensitive data not only violates individuals' privacy rights but can also lead to severe consequences such as identity theft and unauthorized surveillance. Moreover, the rise of algorithmic decision-making raises concerns about bias and fairness. Biases in data or algorithms can perpetuate and even exacerbate existing social inequalities, reinforcing discrimination in areas like employment, finance, and criminal justice.
As society becomes more reliant on data-driven insights, the ethical responsibility to ensure these insights are fair, unbiased, and transparent becomes increasingly urgent. The Need for Ethical Data Science, therefore, encompasses a broader societal awareness of the potential negative impacts of data misuse and a growing demand for responsible, accountable, and transparent data practices.
Key Ethical Challenges in Data Science
In the rapidly evolving landscape of data science, ethical challenges have become central to discussions surrounding responsible and meaningful data use. Among the foremost challenges is the issue of privacy and data protection. As data collection becomes more pervasive, concerns about how personal information is handled, stored, and shared have intensified. The need to strike a delicate balance between extracting valuable insights from data and safeguarding individuals' privacy is a complex challenge that requires careful consideration.
Bias and fairness in algorithms represent another critical ethical challenge. Algorithms, when trained on biased or incomplete datasets, can perpetuate and even exacerbate societal inequalities. This raises questions about the fairness and equity of decision-making processes informed by such algorithms, particularly in areas like hiring, lending, and criminal justice. Addressing bias requires a multidimensional approach involving diverse and representative datasets, transparent model development, and ongoing scrutiny of algorithmic outcomes.
Transparency and accountability pose additional challenges in ethical data science. Many machine learning models operate as complex 'black boxes,' making it difficult to understand how they arrive at specific decisions. Lack of transparency can undermine trust in the technology and its applications. Establishing mechanisms for explaining and auditing algorithmic decisions is crucial for ensuring accountability and providing individuals with insights into the reasoning behind automated choices.
Moreover, the issue of consent and informed data usage adds a layer of complexity to ethical considerations. As data is increasingly collected from various sources, ensuring that individuals are adequately informed about how their data will be used and obtaining meaningful consent becomes a daunting challenge. Striking a balance between obtaining the necessary data for analysis and respecting individuals' autonomy over their personal information is a continuous ethical dilemma.
Approaches to Ethical Data Science
Approaches to Ethical Data Science" involves strategies, methodologies, and frameworks that individuals, organizations, and the broader data science community can adopt to ensure that their data-related practices align with ethical principles and standards. These approaches are essential for mitigating risks associated with data misuse, bias, and privacy violations, and for promoting responsible and transparent data practices. Here are some key explanations for approaches to ethical data science:
Development of Ethical Guidelines and Frameworks
- This approach involves creating comprehensive sets of guidelines, principles, and codes of conduct specifically tailored to ethical data science. These guidelines provide clear standards for data collection, analysis, and usage.
- Organizations and professional associations often develop and promote these frameworks to help data scientists make ethical decisions in their work.
Integration of Ethical Considerations in the Data Science Lifecycle
- Ethical considerations should be woven into every stage of the data science process, from data collection and preprocessing to model development and deployment.
- Data scientists must assess potential ethical issues at each stage and make informed choices to minimize harm and bias.
Role of Interdisciplinary Collaboration:
- Collaboration between data scientists, ethicists, legal experts, and other relevant professionals is crucial for addressing ethical challenges.
- Multidisciplinary teams can provide diverse perspectives and help identify and resolve ethical issues that may not be apparent to data scientists alone.
Ethical Data Collection and Storage
- This approach emphasizes the importance of obtaining informed consent when collecting data from individuals.
- It also involves securely storing data, ensuring that sensitive information is protected from unauthorized access, and complying with data protection regulations.
Implementing Ethical Data Science in Practice
In the ever-evolving field of data science, the need to implement ethical principles has become increasingly vital. The practical implementation of ethical data science involves translating theoretical ethical frameworks into actionable steps that data scientists and organizations can follow in their day-to-day operations. This process encompasses various stages of the data lifecycle, from data collection to analysis, model deployment, and beyond.
One crucial aspect of ethical data science implementation is the responsible collection and storage of data. This begins with obtaining informed consent from individuals whose data is being collected. Organizations must be transparent about how the data will be used and ensure that individuals have the option to opt in or out. Furthermore, data should be securely stored and protected to prevent unauthorized access and breaches, which can have significant ethical implications.
In the development of algorithms and machine learning models, ethical considerations continue to play a pivotal role. Ensuring fairness and reducing bias in algorithms is a top priority. This involves carefully curating training data, regularly auditing algorithms for bias, and adjusting them to minimize unfair outcomes. Achieving fairness is a complex task, as it requires a deep understanding of the potential sources of bias and the application of techniques to mitigate them.
The Role of Data Scientists and Organizations
In the realm of ethical data science, the role of data scientists and organizations is pivotal in shaping responsible practices and ensuring that data-driven decision-making aligns with moral standards. Data scientists, as the architects of algorithms and analytical models, bear the responsibility of integrating ethical considerations at every stage of the data science lifecycle. This involves not only technical proficiency but also a deep understanding of the social, legal, and cultural implications of their work.
Data scientists play a critical role in addressing issues such as bias and fairness in algorithms. They need to be aware of the potential biases in data sources, actively work towards mitigating them, and ensure that the models they create are fair and unbiased. Additionally, transparency and accountability are integral to the ethical use of data. Data scientists should be committed to making their processes transparent, allowing for scrutiny and understanding by stakeholders.
Organizations, on the other hand, hold the responsibility of fostering a culture of ethical data use. This begins with the formulation of clear ethical guidelines and frameworks that guide data scientists in their work. Organizations must prioritize continuous education and training for their data science teams, keeping them abreast of the latest ethical standards and best practices. Moreover, they need to establish mechanisms for ongoing monitoring and evaluation to ensure that ethical considerations remain at the forefront of data-related activities.
The rise of ethical data science signifies a maturation of the field, acknowledging the profound impact that data-driven decisions can have on individuals and society. By embracing privacy, fairness, transparency, informed consent, and social responsibility, ethical data science charts a course towards a future where data is a force for good. As we navigate the moral landscape of data science, it is crucial to recognize that the responsible use of data is not only a professional obligation but a moral imperative that shapes the world we live in.