The Secrets of Machine Learning in Data Analytics
The secrets of machine learning in data analytics with our comprehensive guide. Learn about algorithms, best practices, and avoid common pitfalls. Discover the power of data analysis today!
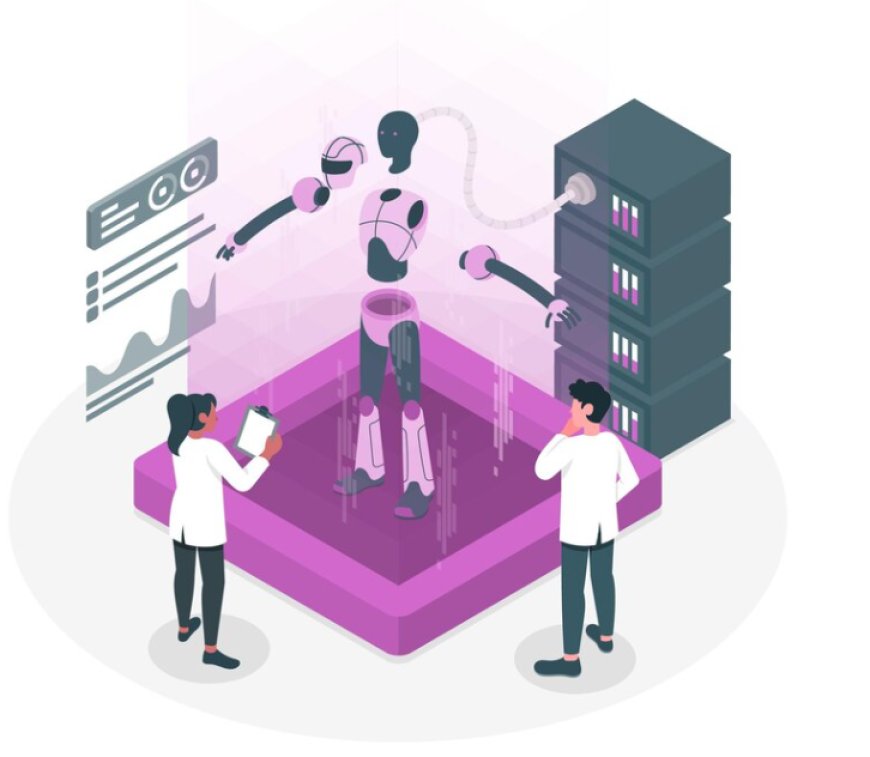
In an age defined by data, the ability to harness its full potential has become a coveted skill in countless industries. Data analytics, the process of extracting insights from vast datasets, has grown exponentially in importance. Yet, lurking beneath the surface of this data-driven revolution lies a secret weapon: machine learning. This powerful technique empowers us to uncover patterns, make predictions, and automate decision-making in ways previously thought impossible.
Data Analytics Landscape: Machine Learning's Role in Taming Big Data
The data analytics landscape has undergone a seismic shift in recent years. With the explosion of data in every imaginable sector, from e-commerce to healthcare, there is a pressing need for advanced tools and techniques to make sense of this data deluge. Machine learning has emerged as a pivotal player in this transformation, offering the capability to extract valuable insights from vast and complex datasets.
In this landscape, machine learning acts as the lighthouse, guiding analysts through the stormy seas of big data. It does so by employing sophisticated algorithms that can detect patterns, trends, and anomalies that would be nearly impossible for human analysts to uncover. Whether it's predicting customer preferences, optimizing supply chains, or diagnosing diseases, machine learning empowers data analytics to reach new heights of efficiency and accuracy.
As we delve deeper into the data analytics landscape, we find that machine learning is not just a tool but a fundamental component, helping organizations make data-driven decisions, improve operations, and gain a competitive edge in a world flooded with information.
Transitioning from Manual Analysis to Machine Learning in Data Analytics
Transitioning from Manual Analysis to Machine Learning in Data Analytics explores the evolving landscape of data analysis, highlighting the challenges and limitations of traditional, manual approaches. In the digital age, the exponential growth of data has made it increasingly difficult for human analysts to effectively process and derive meaningful insights from vast datasets. This transition is necessitated by the need to tackle the complexities of modern data, which can involve unstructured and high-dimensional information.
The article emphasizes that manual analysis, though useful, falls short when dealing with intricate patterns and predicting trends in large-scale datasets. To address these limitations, machine learning has emerged as a powerful tool. By introducing machine learning techniques, organizations can automate data analysis processes, uncover hidden patterns, and make data-driven decisions more efficiently. The transition to machine learning signifies a shift toward greater accuracy, scalability, and the ability to handle diverse and complex data, making it a pivotal step in the evolution of data analytics.
How does machine learning work in data analytics? What are the secrets to successful implementation?
-
How does machine learning work in data analytics?
This question delves into the fundamental principles of how machine learning, a subset of artificial intelligence, is applied in the field of data analytics. Machine learning involves training algorithms to recognize patterns, make predictions, and provide insights from large datasets. It does this through the use of various techniques, including supervised learning, unsupervised learning, and deep learning. By exploring this question, you can explain the underlying mechanisms of machine learning and how it helps in uncovering meaningful information from data.
-
What are the secrets to successful implementation?
Successful implementation of machine learning in data analytics requires careful planning, strategy, and execution. This question invites discussion about the strategies and best practices that lead to effective implementation. Some of the "secrets" may include selecting the right algorithms, collecting high-quality data, ensuring a well-defined problem statement, and iterating the model for continuous improvement. Addressing this question will provide readers with insights into the steps and strategies that can maximize the success of their machine-learning projects.
-
What are the common pitfalls to avoid?
machine learning and data analytics, there are numerous pitfalls that can hinder progress and lead to project failures. This question focuses on identifying these potential pitfalls and discussing ways to avoid them. Common pitfalls may include overfitting, inadequate data preprocessing, lack of domain expertise, or ignoring ethical considerations in data analytics. By addressing this question, you can help readers steer clear of these common mistakes and challenges, ultimately leading to more successful machine learning implementations.
Mastering Machine Learning in Data Analytics: Algorithms, Best Practices, and Pitfalls
How Machine Learning Algorithms Work in Data Analytics
-
This part of the topic delves into the fundamental principles of machine learning algorithms. It explains how these algorithms process and analyze data to make predictions or classifications.
-
It may discuss the different types of machine learning algorithms, such as supervised, unsupervised, and reinforcement learning, and their applications in data analytics.
-
You can also touch upon concepts like data preprocessing, feature selection, model training, and model evaluation, providing a comprehensive understanding of the process.
Best Practices for Successful Machine Learning Implementation
-
This section outlines the strategies and approaches that contribute to the successful implementation of machine learning in data analytics projects.
-
It includes best practices related to data collection, data quality, feature engineering, model selection, and hyperparameter tuning.
-
Discuss the importance of iterative development, cross-validation, and model interpretability in ensuring the reliability and effectiveness of machine learning solutions.
Insights into Common Mistakes and How to Avoid Them:
-
Here, you highlight the pitfalls and mistakes that individuals and organizations often encounter when implementing machine learning in data analytics.
-
Common mistakes may include overfitting, underfitting, data leakage, and the misuse of algorithms. Explain why these mistakes occur and their consequences.
-
Offer insights into how these mistakes can be avoided through careful planning, rigorous testing, and adherence to best practices.
The Future of Machine Learning in Data Analytics
The future of machine learning in data analytics holds great promise and transformational potential. As data continues to proliferate at an unprecedented rate, machine learning will play an even more pivotal role in extracting meaningful insights from the vast ocean of information. One key trend is the democratization of machine learning, making it more accessible to non-experts through user-friendly tools and platforms.
Additionally, there's a growing emphasis on explainable AI, which seeks to make machine learning models more transparent and interpretable, especially in critical domains like healthcare and finance. AutoML is also on the rise, enabling automated model selection and hyperparameter tuning, reducing the barrier to entry for organizations.
Machine learning will contribute to addressing global challenges, such as climate change and healthcare, by enabling more accurate predictions and data-driven decisions. The integration of machine learning with edge computing and IoT devices is set to revolutionize real-time analytics.
The future of machine learning in data analytics is marked by accessibility, interpretability, automation, and its role in solving complex, real-world problems. It's a landscape of exciting opportunities and ongoing innovation.
The power of machine learning in analytics unveils new horizons. Embracing these hidden pathways leads to discovery and transformation, where insights become innovation, and complexity meets clarity. The secrets of machine learning in data analytics are the keys to a brighter future, where patterns and possibilities converge.