Machine Learning: The Future of Intelligence
Explore the transformative impact of Machine Learning (ML) on diverse industries, from healthcare to user experience and autonomous systems. Delve into its potential to redefine intelligence, address current challenges, and navigate ethical considerations.
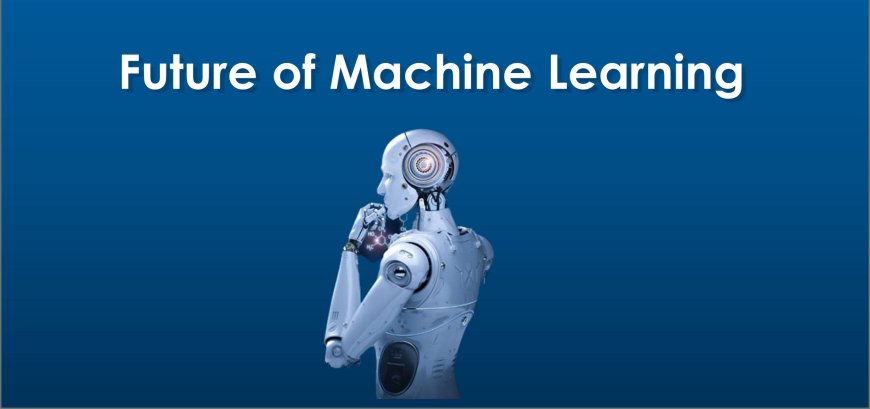
Machine Learning (ML) is an evolving field in technology that is a key driver of innovation and change. ML is more than just a new technology; it plays a major part in determining how intelligence develops in the future. Examining its subtleties exposes a complex environment in which ML's adaptable skills impact several industries. Its influence transcends the technology sphere, making it a crucial driver in shaping the intelligence environment of the future.
ML's Versatility: Shaping Diverse Industries
Machine Learning (ML), a branch of artificial intelligence, has experienced remarkable expansion in recent years. The capacity of machines to learn from data and enhance their capabilities has unlocked new possibilities across diverse fields. Whether in healthcare, finance, marketing, or entertainment, ML has demonstrated its adaptability, offering a pragmatic approach to problem-solving. Its potential to reshape traditional problem-solving methods underscores its role as a versatile tool, contributing to advancements in practical applications across various industries.
Balancing Challenges and Potential in Machine Learning
Despite notable progress, Machine Learning faces hurdles like the requirement for extensive datasets, ethical concerns, and the challenge of interpreting models. Researchers and practitioners are actively working to overcome these obstacles. It's crucial to acknowledge these challenges, yet not let them overshadow the substantial potential ML offers. By addressing these issues with diligence, the field can continue its positive trajectory, contributing meaningfully to various domains without downplaying the complexities inherent in its application.
How will Machine Learning continue to shape the future of intelligence, and what role will it play in addressing current challenges?
Machine Learning (ML) is set to redefine intelligence by advancing healthcare diagnostics, refining user experiences through recommendation systems, powering autonomous systems, and revolutionizing natural language processing. As ML's influence expands, it addresses challenges such as ethical concerns through initiatives like explainable AI. The ongoing integration of ML in various domains positions it as a key player in shaping a more intelligent and responsive future.
Let’s take a look at each type in a little more depth.
1. Innovations in Healthcare
Machine Learning applications in healthcare are transforming the industry. Through the analysis of extensive datasets, ML algorithms can identify patterns and correlations that might be imperceptible to human observers. This capability is particularly valuable in diagnostics, where early detection is crucial. Machine Learning models can process medical images, such as X-rays and MRIs, to identify potential health issues at an early stage. Moreover, personalized medicine is becoming a reality as ML algorithms analyze genetic data to tailor treatment plans based on an individual's unique genetic makeup. In drug discovery, ML accelerates the identification of potential drug candidates, reducing the time and cost traditionally associated with this process.
2. Enhancing User Experience
ML algorithms are the driving force behind the personalized experiences users encounter on various platforms. Streaming services leverage ML to understand user preferences and recommend content, creating a more engaging and tailored viewing experience. E-commerce websites utilize ML algorithms for personalized product recommendations, enhancing user satisfaction and increasing conversion rates. As these algorithms evolve, they not only improve recommendations but also contribute to the efficient use of resources by reducing unnecessary data transfer and processing power.
3. Autonomous Systems
The integration of Machine Learning in autonomous systems is revolutionizing transportation. Self-driving cars and drones equipped with ML algorithms learn from real-world scenarios, continuously improving their decision-making capabilities. In the context of self-driving cars, ML enables vehicles to navigate complex and dynamic environments by recognizing patterns in real-time data from sensors. This technology has the potential to enhance road safety, reduce accidents, and optimize traffic flow. The adaptability of autonomous systems driven by ML makes them well-suited for a variety of applications, from last-mile delivery drones to public transportation.
4. Natural Language Processing (NLP)
NLP, a subfield of Machine Learning, focuses on the interaction between computers and human language. In language translation, ML models powered by NLP have achieved remarkable accuracy, breaking down language barriers and facilitating global communication. Sentiment analysis, another application of NLP, allows businesses to gauge public opinion and customer feedback, informing decision-making processes. Chatbots, equipped with NLP capabilities, provide efficient and human-like interactions in customer service, improving response times and user satisfaction. The advancements in NLP contribute not only to improved communication but also foster collaboration on a global scale.
5. Ethical Considerations
As Machine Learning becomes increasingly integrated into various aspects of society, ethical considerations have become paramount. Issues such as bias in algorithms, data privacy, and the accountability of AI systems are gaining prominence. The industry is actively addressing these concerns by developing frameworks for fairness and transparency. Explainable AI, an initiative within ML, aims to make machine learning models more interpretable, addressing the "black-box" nature of some algorithms. By promoting ethical practices, the goal is to ensure that the benefits of ML are distributed equitably and that potential risks are mitigated responsibly. This ongoing dialogue around ethics reflects a commitment to responsible AI development and deployment.
How is machine learning related to AI?
Machine learning (ML) is a subset of artificial intelligence (AI), and the relationship between the two can be understood as follows:
Definition of Artificial Intelligence
-
AI: Artificial Intelligence refers to the development of computer systems that can perform tasks that typically require human intelligence. These tasks include problem-solving, learning, speech recognition, decision-making, and language translation.
Scope of AI
-
Broad Field: AI is a broad field that encompasses various approaches and techniques to mimic or simulate human intelligence. It includes both rule-based systems (traditional programming) and learning-based systems (machine learning).
Machine Learning within AI
-
Subset: Machine Learning is a subset of AI that focuses on developing algorithms and models that allow computers to learn from data. Instead of being explicitly programmed to perform a task, ML systems are trained using large datasets, allowing them to improve their performance over time.
Learning from Data
-
Data-Driven Approach: While traditional AI methods involve explicit programming to define rules and decision-making processes, machine learning adopts a data-driven approach. ML algorithms use statistical techniques to identify patterns and make predictions or decisions without being explicitly programmed for each scenario.
Types of Machine Learning
-
Supervised Learning: In this type of ML, the algorithm is trained on labeled data, where the input-output pairs are provided. It learns to make predictions or decisions based on the patterns observed in the training data.
-
Unsupervised Learning: Here, the algorithm is given unlabeled data and must find patterns or relationships within the data on its own. Clustering and dimensionality reduction are common tasks in unsupervised learning.
-
Reinforcement Learning: This involves training a model to make sequences of decisions by interacting with an environment. The model receives feedback in the form of rewards or penalties, allowing it to learn optimal strategies.
AI Applications
-
AI Encompasses More: While machine learning is a powerful tool within AI, the broader field of AI includes other approaches, such as expert systems, knowledge representation, planning, and natural language processing. These areas contribute to creating intelligent systems with diverse capabilities.
Interconnected Evolution
-
Feedback Loop: Machine learning, within the broader context of AI, has led to a feedback loop where advances in ML techniques contribute to enhancing AI capabilities. As ML algorithms become more sophisticated and capable, they drive advancements in AI applications and systems.
Machine Learning's evolution within the broader framework of Artificial Intelligence is reshaping industries and addressing challenges. From healthcare innovations to enhancing user experiences and navigating ethical considerations, ML's versatility positions it as a pivotal force in shaping the future of intelligence. As ethical guidelines and technological advancements converge, the trajectory of ML promises a more intelligent and responsible future.