The Key Domains of Artificial Intelligence: Comprehensive Overview
Explore the key domains of Artificial Intelligence in this comprehensive overview. Learn about AI's applications, challenges, and prospects.
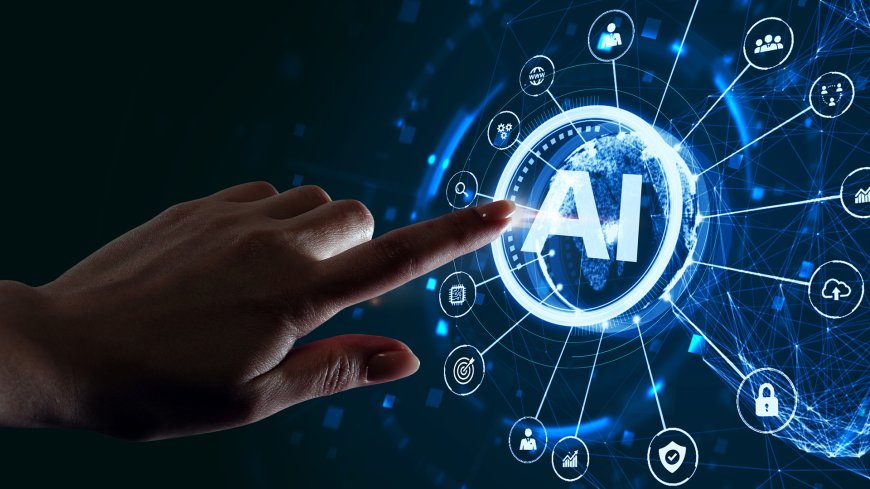
Artificial intelligence (AI) is like teaching computers to think and learn on their own, similar to how humans do. It's about making machines smart enough to understand information, solve problems, and even make decisions without constant human guidance. Think of it as giving computers the ability to learn from data and experiences, just like we learn from our surroundings.
The cool thing about AI is how it can make our lives easier and more efficient. From helping doctors diagnose diseases faster to making our daily commute smoother with self-driving cars, AI is all about using technology to solve everyday problems. It's like having a super-smart assistant who can handle complex tasks and make our world a bit smarter and more connected.
The Scope of Artificial Intelligence
AI is changing how industries work, like healthcare, finance, and transportation, by making processes smoother and sparking new ideas. In healthcare, it helps doctors diagnose faster and tailor treatments. Finance benefits from AI spotting fraud and boosting investments. Self-driving cars, thanks to AI, enhance safety on the roads. From customer service to manufacturing, AI's presence is reshaping how businesses function, adding value. However, AI brings up questions about jobs and ethics. Though it promises new job openings and better living standards, it also raises worries about job loss and fairness. The future of AI depends on how we handle its growth and integrate it into society, ensuring it benefits everyone while addressing any issues along the way.
What are the domains of Artificial Intelligence?
Artificial intelligence domains encompass machine learning, natural language processing, robotics, computer vision, and expert systems. These domains involve developing algorithms and systems that enable computers to perform tasks typically requiring human intelligence.
Natural Language Processing (NLP)
Natural Language Processing (NLP) teaches computers to understand and interact with human language and is used in translation, sentiment analysis, and virtual assistants like Siri or Alexa. In customer service, NLP responds to messages, aids search engines in understanding queries, and helps analyze medical records in healthcare. Chatbots use NLP for instant support, while voice recognition enables hands-free communication.
Machine Learning (ML)
Machine learning (ML) is teaching computers to learn from data and make decisions without explicit instructions. It's done through algorithms, helping machines get better at tasks through experience. Types include supervised learning, where labeled data teaches, like spotting spam emails; unsupervised learning, which finds patterns in unlabeled data, like grouping similar items; and reinforcement learning, where trial and error, like navigating a maze, guides learning with rewards or penalties, often using algorithms like Q-learning.
Computer Vision:
Computer vision is a field of artificial intelligence that teaches computers to interpret and understand visual information from images or videos. It enables machines to recognize objects, scenes, and gestures, similar to how humans perceive the world around them.
Computer vision is applied in healthcare for medical imaging and surgery, in retail for cashier-less stores and inventory management, in automotive for self-driving cars and safety features, and security for facial recognition and surveillance.
Ensuring safety is crucial as robots work alongside humans, requiring design with built-in safety features, effective risk assessment, and proper operator training. Concerns about job displacement arise with automation, demanding education and training programs to help workers adapt and find new opportunities. Ethical use of robotics involves guidelines to ensure responsible behavior, especially in sensitive sectors like healthcare and the military, addressing privacy, autonomy, and accountability concerns.
Robotics
Robotics involves creating and programming robots to work independently, focusing on smart machines capable of sensing and interacting with their surroundings. They find applications in manufacturing, healthcare, agriculture, and space exploration, streamlining processes and enhancing efficiency.
However, ethical considerations such as ensuring robot safety, addressing job displacement concerns, and developing guidelines for ethical robot behavior arise. There should be steps to avoid errors, workers impacted by automation must participate in educational programs, and ethical practices are essential—particularly in delicate fields like healthcare and military applications.
Expert Systems
Expert Systems are computer programs that imitate human decision-making in specific fields, using knowledge bases and inference engines to provide advice or solutions. They're applied in healthcare, finance, and technical support, aiding in diagnosis, analysis, and problem-solving. Despite their usefulness, they struggle with uncertainty and require constant knowledge base maintenance.
Future directions may see expert systems advancing with AI and machine learning, enhancing their ability to handle complex tasks and uncertainties. Integration with big data and natural language processing could further improve accuracy and usability, expanding their applications across various industries.
Reinforcement Learning
Reinforcement Learning is a method where a computer learns by trial and error to achieve goals, receiving feedback in the form of rewards or penalties. It's used in robotics for tasks like navigation, gaming for intelligent game agents, and recommendation systems for personalized content suggestions.
Challenges in reinforcement learning include handling complex environments, improving efficiency with less data, and addressing safety and ethical concerns in real-world applications like autonomous vehicles or healthcare. Ongoing research focuses on enhancing algorithms to overcome these obstacles and expand the scope of reinforcement learning.
Interdisciplinary Aspects of AI Domains
The interdisciplinary nature of AI involves its connection with other technologies like IoT and blockchain, creating opportunities for smart homes, autonomous vehicles, and supply chain management. Ethical and societal implications across AI domains raise concerns about privacy, bias, and job displacement, urging collaboration to develop regulations and guidelines for responsible AI use.
Collaborative opportunities and future trends in AI domains promise innovation in healthcare, finance, and education. Integrating AI with emerging technologies like augmented reality and quantum computing could address complex challenges and drive societal progress. Embracing collaboration and addressing ethical concerns will shape the future of AI domains positively.
Career Paths and Opportunities in AI Domains
Roles and Responsibilities
-
Data Scientist: Analyzes large datasets using statistical techniques to extract insights and build predictive models to inform decision-making processes.
-
Machine Learning Engineer: Develops and deploys machine learning algorithms to solve real-world problems, such as image recognition, natural language processing, and recommendation systems.
-
AI Research Scientist: Conducts research to advance AI technologies by developing new algorithms, improving existing models, and exploring innovative applications.
-
AI Ethicist: Ensures the ethical use of AI technologies by addressing societal implications and advocating for fairness, transparency, and accountability in AI systems.
-
Robotics Engineer: Designs and develops robots for automation and autonomous systems, including tasks like robot motion planning, control, and perception.
-
Natural Language Processing (NLP) Engineer: Builds systems that understand and generate human language, enabling applications such as chatbots, language translation, and sentiment analysis.
Skills and Educational Background
Proficiency in programming languages like Python, R, or Java, along with strong mathematical and statistical skills, is essential. A background in computer science, engineering, or related fields is common, with advanced degrees (master's or Ph.D.) beneficial for roles involving research or specialized expertise. Additionally, skills in data analysis, machine learning algorithms, and domain knowledge in specific AI applications are valuable. While specific cut-off mark percentages may vary depending on the institution and program, a solid academic background in STEM subjects is typically required for admission into AI-related degree programs or positions.
Career Trends and Job Market Outlook
The demand for AI professionals is growing rapidly across industries, offering diverse career opportunities. Emerging trends include the integration of AI with other technologies like IoT and blockchain, leading to new roles and applications. The job market outlook is promising, with high demand for AI talent expected to continue as organizations invest in AI-driven innovation and automation. Keeping abreast of emerging technologies and acquiring relevant skills will be crucial for success in the dynamic field of AI.
Challenges and Considerations in AI Development:
a. Ethical and Bias Concerns: Ensuring fairness and transparency in AI decision-making is crucial, as AI systems may unintentionally inherit biases from the data they're trained on, leading to unfair outcomes for certain groups.
b. Data Privacy and Security Issues: Protecting data privacy and security is vital, as AI systems rely on vast amounts of data, posing risks of unauthorized access, misuse, or breaches that could undermine trust in AI technologies.
c. Regulatory and Legal Challenges: Navigating the regulatory landscape for AI involves addressing issues like liability for AI-related errors, establishing standards for development and deployment, and ensuring compliance with international regulations without stifling innovation.
Future Trends in AI Domains:
Expect continued advancements in AI technologies, such as improved machine learning algorithms, enhanced natural language processing capabilities, and more sophisticated robotics systems. Anticipate potential disruptions and innovations in various sectors, including healthcare, finance, and transportation. Examples include personalized medicine, AI-driven financial services, and autonomous vehicles reshaping industries.
Predictions for the future of AI include increased integration with other technologies like IoT and blockchain, enabling new applications and opportunities. Additionally, expect AI to play a significant role in addressing global challenges such as climate change and healthcare access.
We've covered key points in AI domains, including roles like data scientist and machine learning engineer, and challenges such as ethical concerns and data privacy issues. The diversity and impact of AI across industries, from healthcare to finance, highlight its potential to revolutionize how we work and live. As AI continues to advance, its integration with other technologies and its role in addressing global challenges will shape the future landscape, offering both opportunities and considerations for society as a whole.