How AI is Reshaping the Future of Economics
Explore the transformative impact of AI on the future of economics. From data-driven decision-making to personalized economic experiences, discover how artificial intelligence is reshaping economic landscapes.
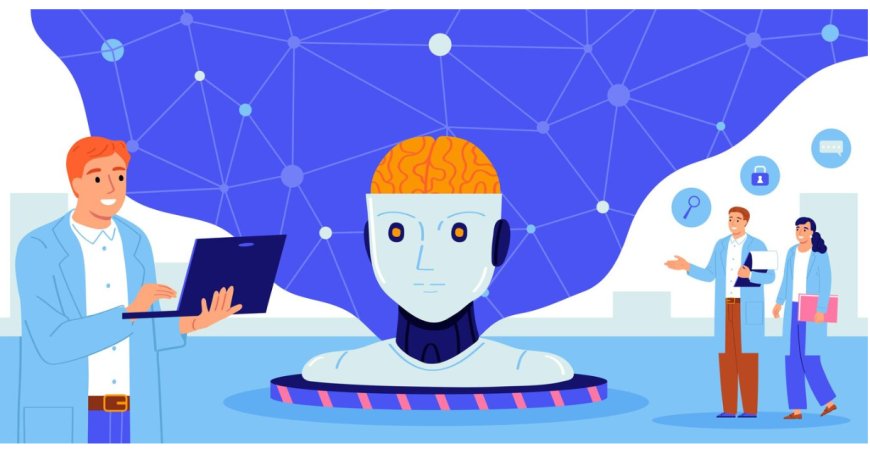
The 21st century has witnessed an unprecedented convergence of technology and economics, with Artificial Intelligence (AI) emerging as the driving force behind transformative changes. In this era of rapid innovation, AI is not just a technological advancement but a catalyst reshaping the very fabric of economies worldwide. From predictive analytics to automated decision-making processes, AI is leaving an indelible mark on economic landscapes.
Data-Driven Decision Making
In the ever-evolving landscape of economics, the emergence of data-driven decision-making stands out as a pivotal development. At its core, this approach relies on harnessing the power of data to inform and guide the decision-making process. Unlike traditional methods that might rely on intuition or past experiences alone, data-driven decision-making leverages advanced analytics and Artificial Intelligence (AI) algorithms to scrutinize vast datasets. By extracting meaningful patterns, correlations, and trends from this sea of information, decision-makers gain valuable insights into market dynamics, consumer behaviors, and overall economic trends.
The significance of data-driven decision-making lies in its ability to replace gut instincts with evidence-based conclusions. This method enables policymakers, business leaders, and economists to make more informed and objective choices. For instance, in economic policy formulation, the analysis of historical data and real-time indicators allows for a deeper understanding of the impact of decisions on various sectors. This, in turn, enhances the precision and effectiveness of economic strategies.
Moreover, the advent of Big Data technologies and AI has catapulted data-driven decision-making to new heights of sophistication. Advanced algorithms can process and analyze immense datasets in real-time, offering decision-makers a dynamic and up-to-the-minute understanding of economic conditions. This agility is particularly crucial in today's fast-paced and interconnected global economy.
Predictive Analytics
Predictive analytics is a powerful subset of data analytics that involves the use of advanced statistical and machine-learning techniques to forecast future outcomes or trends based on historical data. It goes beyond simply describing past events; instead, it focuses on making informed predictions about what is likely to happen in the future. Here are some key points to understand about predictive analytics
-
Data-Driven Insights: At its core, predictive analytics relies on data. It takes historical data, which can include a wide range of variables and factors, and uses this information to identify patterns and relationships that can be used to make predictions. This data can come from various sources, such as customer records, financial transactions, sensor data, or any dataset with a time element.
-
Machine Learning Algorithms: Predictive analytics often employs machine learning algorithms to analyze data. These algorithms are capable of learning from historical data and making predictions based on that learning. They can handle large and complex datasets, making them well-suited for tasks like forecasting sales, predicting equipment failures, or even identifying potential health risks.
-
Applications Across Industries: Predictive analytics finds applications in numerous industries, including finance, healthcare, marketing, manufacturing, and more. For example, in finance, it can be used to predict stock prices or identify fraudulent transactions. In healthcare, it can help predict patient outcomes or disease outbreaks. Its versatility means that it can be tailored to address a wide range of business and societal challenges.
-
Predictive Modeling: To perform predictive analytics, analysts create predictive models. These models are mathematical representations of the relationships between variables in the data. The process typically involves data preprocessing, feature selection, model training, and evaluation. The goal is to build a model that can make accurate predictions on new, unseen data.
-
Continuous Learning: Predictive analytics is not a one-time task; it's an ongoing process. As new data becomes available, the models can be updated and refined to improve accuracy. This adaptability is especially valuable in industries where conditions change rapidly, such as e-commerce, where customer preferences evolve.
Financial Modeling and Algorithmic Trading
In the fast-paced world of finance, the integration of Artificial Intelligence (AI) has ushered in a new era characterized by advanced financial modeling and algorithmic trading. This paradigm shift is redefining how financial professionals analyze markets, make investment decisions, and execute trades. At the heart of this transformation is the capability of AI to process vast amounts of financial data with unprecedented speed and accuracy.
Financial modeling, traditionally a labor-intensive and time-consuming process, has been streamlined by AI algorithms. These algorithms can analyze historical market data, economic indicators, and even social media sentiments to create sophisticated models that forecast market trends and assess risk. The result is a more nuanced understanding of market dynamics, enabling investors and financial institutions to make informed decisions in real time.
Algorithmic trading, another cornerstone of this transformation, relies on AI to execute trades with incredible speed and efficiency. These algorithms can react to market fluctuations in milliseconds, far surpassing the capabilities of human traders. The automation of trading processes not only minimizes the risk of emotional decision-making but also ensures that trades are executed at optimal moments, maximizing returns.
Automation of Routine Tasks
Automation of routine tasks is a transformative aspect of AI's impact on economics, redefining the nature of work and productivity. In various economic sectors, AI is revolutionizing how routine and repetitive tasks are handled, freeing up human resources for more strategic and creative endeavors. The implementation of AI-driven automation is particularly evident in fields such as accounting, data entry, and basic analysis, where algorithms can perform tasks with precision and efficiency unmatched by human counterparts.
One of the key advantages of automation in routine tasks is its potential to enhance efficiency and reduce costs. AI systems can tirelessly process vast amounts of data, execute repetitive tasks with minimal error, and operate around the clock without the need for breaks or rest. This not only accelerates the pace of work but also allows businesses to allocate human resources to tasks that demand critical thinking, problem-solving, and emotional intelligence.
However, the rise of automation also poses challenges, such as concerns about job displacement and the need for upskilling the workforce. While routine tasks are automated, there is an increasing demand for individuals skilled in developing, maintaining, and overseeing AI systems. The shifting employment landscape requires proactive measures in education and workforce development to ensure that individuals are equipped with the skills necessary for the jobs of the future.
Moreover, the benefits of automation extend beyond mere efficiency gains. By automating routine tasks, businesses can achieve higher levels of accuracy and consistency, reducing the likelihood of errors that may have financial or operational implications. This, in turn, contributes to improved overall quality and reliability in various economic processes.
Enhanced Resource Allocation
Enhanced resource allocation, in the context of AI and economics, refers to the use of advanced algorithms and data-driven insights to optimize the distribution and utilization of resources within an economy. This process involves analyzing large datasets, identifying patterns, and making informed decisions to ensure that resources, such as budgetary funds, workforce, and materials, are allocated most efficiently and effectively. Here's a more detailed explanation:
-
Data-Driven Decision-Making
Enhanced resource allocation relies on data-driven decision-making. AI algorithms are capable of processing vast amounts of data from diverse sources, including market trends, consumer behavior, and internal organizational data. By analyzing this information, algorithms can identify patterns and trends that might not be immediately apparent to human decision-makers.
-
Optimizing Budget Allocation
In economic management, budgets are crucial tools for allocating financial resources. AI can analyze historical spending patterns, economic indicators, and other relevant data to optimize budget allocations. This ensures that funds are directed toward initiatives that are likely to yield the highest return on investment or address pressing economic needs.
-
Supply Chain Optimization
For industries involved in manufacturing and distribution, efficient supply chain management is essential for success. AI can optimize supply chain processes by analyzing data related to production schedules, inventory levels, transportation logistics, and demand forecasting. This results in a streamlined supply chain that minimizes costs and maximizes efficiency.
Personalized Economic Experiences
In the Artificial Intelligence era, personalized economic experiences have emerged as a transformative force, reshaping how individuals interact with and participate in economic activities. At its core, this paradigm shift is fueled by the ability of AI algorithms to analyze vast amounts of personal data, allowing businesses and financial institutions to tailor their offerings to the unique needs, preferences, and behaviors of individual consumers.
One key aspect of personalized economic experiences is the revolution in marketing strategies. AI-driven algorithms can sift through immense datasets, extracting valuable insights into consumer behavior, purchasing patterns, and preferences. With this information, businesses can create targeted and highly personalized marketing campaigns. From recommending products based on past purchases to delivering personalized promotions, AI ensures that consumers receive messages that resonate with their tastes, increasing the likelihood of engagement and conversion.
In personal finance, AI plays a pivotal role in providing tailored financial advice and services. Machine learning algorithms can analyze an individual's financial history, spending habits, and investment preferences to offer personalized recommendations. This empowers consumers to make more informed financial decisions and ensures that financial products and services align with their unique goals and circumstances.
As we stand at the crossroads of technological innovation and economic evolution, the impact of AI on the future of economics is undeniable. From data-driven decision-making to predictive analytics and personalized economic experiences, AI is fundamentally transforming how economies operate. While the benefits are numerous, it's crucial to navigate this transformative landscape with ethical considerations, ensuring that the power of AI is harnessed responsibly for the benefit of society as a whole. As AI continues to evolve, its role in shaping the future of economics will grow, opening new frontiers and opportunities that will redefine how we understand and participate in the global economy.