The Role of AI in Shaping the Future of Data Analytics
Explore the transformative impact of Artificial Intelligence on data analytics. Discover how AI is reshaping the future of data-driven decision-making, predictive analytics, and personalized customer experiences.
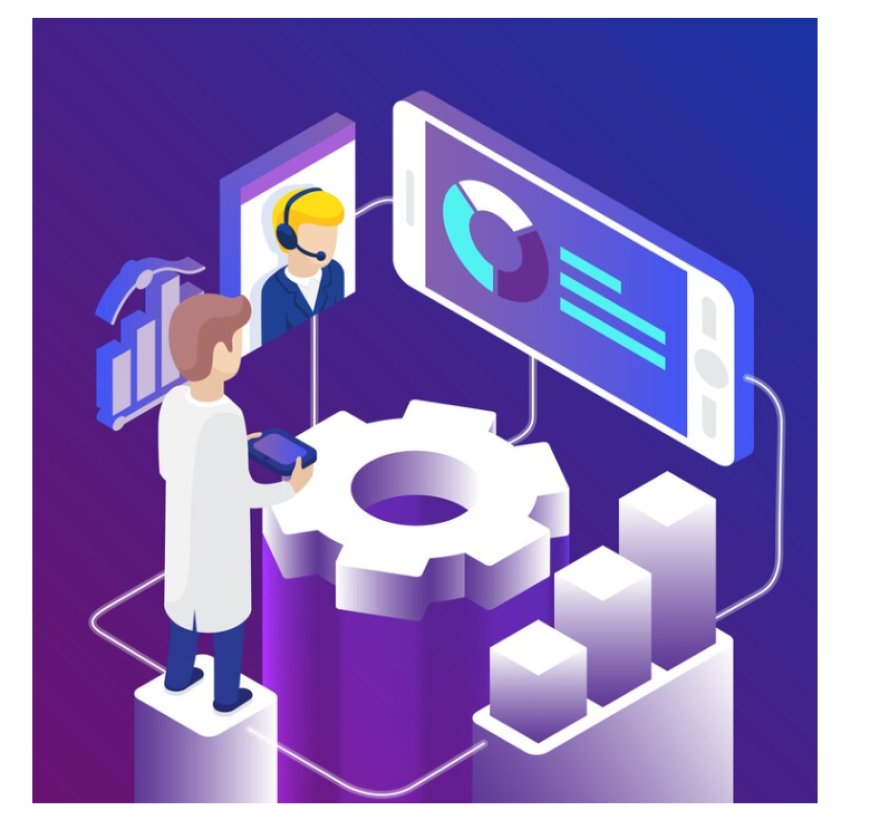
In the era of unprecedented data growth, businesses and organizations are faced with the challenge of extracting meaningful insights from massive datasets. Traditional data analytics methods are often insufficient to handle the sheer volume and complexity of contemporary data sources. This is where Artificial Intelligence (AI) steps in, revolutionizing the landscape of data analytics and paving the way for a more insightful and efficient future.
Unleashing the Power of Big Data
In the digital age, the term "Big Data" has become synonymous with the colossal volumes of information generated daily by a myriad of sources. From social media interactions and online transactions to sensor data and enterprise records, the sheer scale and complexity of this data pose both a challenge and an opportunity. Enter Artificial Intelligence (AI), a revolutionary force that has transformed the way we harness and leverage Big Data.
At its core, Big Data represents a treasure trove of insights waiting to be unearthed. Traditional analytics tools often struggle to process and analyze these vast datasets within reasonable timeframes, leading to the underutilization of valuable information. This is where AI shines. By employing sophisticated algorithms, machine learning models, and deep learning techniques, AI has the capacity to rapidly and intelligently process massive datasets. The ability to discern patterns, correlations, and trends within these data sets enables organizations to extract actionable insights that drive strategic decision-making.
Moreover, the integration of AI with Big Data analytics transcends mere speed and efficiency. AI algorithms can uncover intricate relationships within the data that might elude conventional analysis methods. This capability is especially crucial in fields such as healthcare, finance, and scientific research, where nuanced patterns and associations can hold the key to groundbreaking discoveries or predictive insights.
Predictive Analytics for Informed Decision-Making
Predictive analytics is a data-driven methodology that utilizes statistical algorithms, machine learning, and AI techniques to make predictions about future events or trends based on historical and current data. It plays a pivotal role in informed decision-making across various domains, from business and finance to healthcare and marketing. Here's a more detailed explanation of the concept:
-
Data Utilization
Predictive analytics starts with the collection and analysis of data. It takes historical data as input, which may include information about past events, transactions, customer behavior, or any other relevant factors. This data is used to build predictive models.
-
Building Predictive Models
Predictive models are constructed using a wide array of statistical and machine-learning techniques. These models aim to capture patterns, relationships, and correlations within the data. Algorithms, such as regression analysis, decision trees, neural networks, and more, are applied to the historical data to develop these models.
-
Training and Testing
The predictive models are trained on a subset of the historical data and tested on another subset to ensure their accuracy and reliability. This process helps in refining the models and optimizing their predictive capabilities.
-
Forecasting Future Events
Once the models are developed and validated, they are used to make predictions about future events. For instance, in business, predictive analytics can be used to forecast sales, customer demand, or even equipment failures. These predictions are based on the learned patterns and relationships in the data.
-
Informed Decision-Making
Predictive analytics plays a crucial role in informed decision-making by providing actionable insights. Decision-makers use the predictions to anticipate future scenarios and plan accordingly. For example, a retailer might use predictive analytics to optimize inventory levels, ensuring that products are available when customers demand them but avoiding overstocking.
Automation of Data Processing
In the rapidly evolving landscape of data analytics, the automation of data processing stands out as a key technological advancement. This concept revolves around employing artificial intelligence (AI) and machine learning algorithms to streamline and expedite the various stages of data handling. Data processing encompasses tasks such as data cleaning, transformation, and integration, which are traditionally labor-intensive and time-consuming when done manually.
Automating data processing brings about a paradigm shift in how organizations manage and utilize their data. The primary advantage lies in the acceleration of analytical workflows. AI algorithms are capable of swiftly and accurately handling large datasets, ensuring that insights are generated promptly. This efficiency not only saves valuable time but also allows businesses to make more informed decisions in real time, keeping pace with the dynamic nature of today's markets.
Moreover, the automation of data processing significantly reduces the risk of human error. In manual data processing, there is always the potential for mistakes during repetitive and detail-oriented tasks. Automation minimizes these risks, enhancing the overall reliability of the insights derived from the data. This reliability is crucial for decision-makers who rely on accurate and precise information to guide their strategies.
Natural Language Processing (NLP) for Enhanced Communication
Natural Language Processing (NLP) is a revolutionary aspect of artificial intelligence that focuses on bridging the communication gap between humans and machines. Through NLP, computers can understand, interpret, and generate human-like language, making data analytics tools more accessible to a broader audience. This technology allows users to interact with data analytics platforms using natural language queries, significantly simplifying the process for those without a technical background.
By breaking down the barriers of technical jargon, NLP facilitates more intuitive and user-friendly interactions with data, fostering enhanced communication and collaboration across diverse teams within an organization. The integration of NLP in data analytics not only accelerates decision-making processes but also democratizes data access, empowering individuals to derive meaningful insights without the need for specialized expertise.
Personalized Customer Experiences
Natural Language Processing (NLP) is a revolutionary aspect of artificial intelligence that focuses on bridging the communication gap between humans and machines. Through NLP, computers can understand, interpret, and generate human-like language, making data analytics tools more accessible to a broader audience. This technology allows users to interact with data analytics platforms using natural language queries, significantly simplifying the process for those without a technical background.
By breaking down the barriers of technical jargon, NLP facilitates more intuitive and user-friendly interactions with data, fostering enhanced communication and collaboration across diverse teams within an organization. The integration of NLP in data analytics not only accelerates decision-making processes but also democratizes data access, empowering individuals to derive meaningful insights without the need for specialized expertise.
Fraud Detection and Security
In the fast-paced digital landscape, where transactions and interactions occur at lightning speed, the need for robust fraud detection and security measures has never been more critical. Businesses and organizations face the constant threat of cybercriminals seeking to exploit vulnerabilities for financial gain or other malicious purposes. This is where Artificial Intelligence (AI) emerges as a formidable ally in fortifying the defenses against fraud and ensuring the security of sensitive data.
-
Detecting Anomalies with Machine Learning
AI, particularly machine learning algorithms, is exceptionally adept at recognizing patterns and anomalies within large datasets. In the context of fraud detection, these algorithms analyze historical data to identify irregularities or deviations from normal behavior. For instance, they can detect unusual transaction patterns, login activities, or deviations from established user behavior, signaling a potential security threat.
-
Real-time Monitoring for Swift Response
Traditional fraud detection methods often involve manual review processes that can be time-consuming and reactive. AI-driven systems, on the other hand, enable real-time monitoring of transactions and activities. This proactive approach allows for swift responses to potential security breaches, reducing the window of vulnerability and mitigating the impact of fraudulent activities.
-
Behavioral Biometrics and User Authentication
AI technologies are increasingly leveraging behavioral biometrics for user authentication. This involves analyzing unique patterns in user behavior, such as keystroke dynamics, mouse movements, or touch interactions on mobile devices. By continuously monitoring these behavioral traits, AI systems can detect unauthorized access attempts, even if the login credentials appear valid.
-
Integration of Predictive Analytics
Predictive analytics, powered by AI, plays a pivotal role in fraud prevention. By analyzing historical data, these systems can predict potential future threats and vulnerabilities. This proactive approach allows organizations to implement preemptive security measures, staying one step ahead of cybercriminals who are constantly evolving their tactics.
Continuous Learning and Adaptation
In the realm of artificial intelligence, continuous learning and adaptation represent a paradigm shift in the way machines process information and make decisions. Unlike traditional programs with fixed instructions, AI systems designed for continuous learning have the ability to evolve and improve over time. This concept mirrors the human learning process, where experience leads to enhanced knowledge and better decision-making.
Continuous learning in AI involves feeding new data into existing models, allowing them to update and refine their understanding of patterns and relationships. Machine learning algorithms, a subset of AI, excel at this iterative learning process. As more data becomes available, these algorithms adjust their parameters, optimizing their performance and accuracy. This adaptability is particularly crucial in dynamic environments where patterns and trends may change over time.
Adaptation in AI extends beyond just updating models; it encompasses the ability to respond effectively to new and unforeseen challenges. An adaptive AI system can modify its behavior or predictions based on changes in the data distribution or the introduction of novel inputs. This characteristic is especially valuable in real-world scenarios where conditions are not static, enabling AI systems to maintain relevance and effectiveness.
The significance of continuous learning and adaptation is evident in various AI applications. For instance, in natural language processing, models can improve their language understanding by exposure to diverse linguistic contexts. In autonomous vehicles, continuous learning enables the system to adapt to different driving conditions and scenarios, enhancing safety and performance. Businesses benefit from adaptive AI in areas like customer service, where models can evolve to better understand and respond to the unique preferences and behaviors of individual customers.
The role of AI in shaping the future of data analytics is transformative. From unlocking the potential of Big Data to enabling predictive analytics and automating processes, AI is revolutionizing how organizations derive insights from their data. As we move forward, a thoughtful and ethical integration of AI in data analytics will be essential to harness its full potential while addressing the challenges and ensuring a positive impact on society. Embracing the power of AI in data analytics is not just a technological advancement; it's a strategic imperative for businesses aiming to thrive in the data-driven landscape of the future.