The Role of Computer Vision in Business Intelligence
Explore the pivotal role of computer vision in business intelligence. Learn how this cutting-edge technology enhances data analysis, streamlines decision-making, and revolutionizes insights for smarter business strategies.
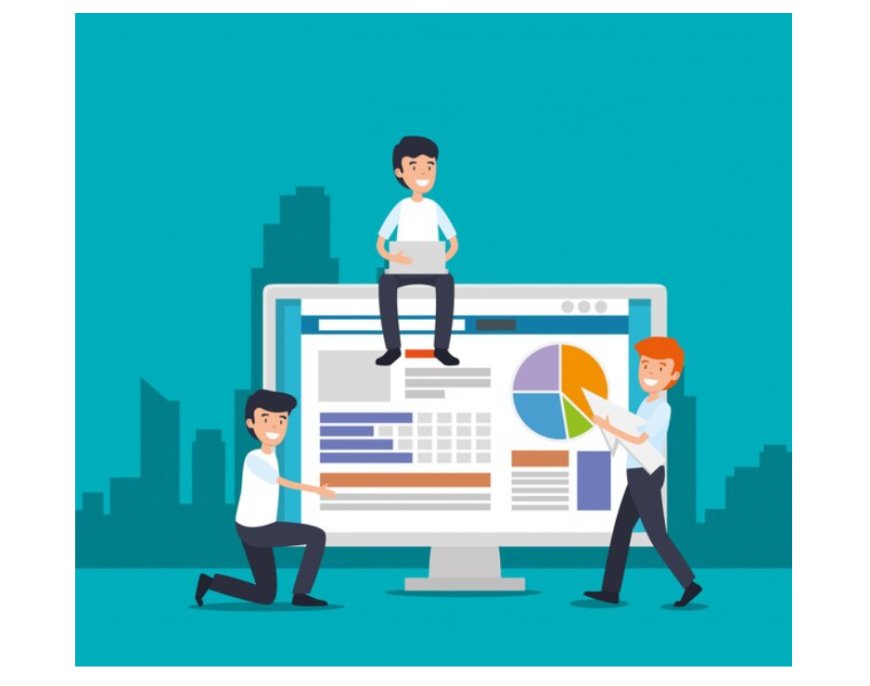
In the dynamic landscape of modern business, staying ahead of the competition requires harnessing the power of cutting-edge technologies. One such revolutionary technology that has been transforming the business landscape is Computer Vision. Often associated with artificial intelligence, Computer Vision empowers machines to interpret and make decisions based on visual data, a capability that is reshaping the realm of Business Intelligence (BI).
Understanding Computer Vision
Computer Vision is a multidisciplinary field of artificial intelligence and computer science that aims to enable machines to interpret and understand visual information from the world, much like the human visual system. It involves the development of algorithms, models, and techniques that allow computers to process, analyze, and make sense of visual data, such as images and videos. This technology has far-reaching applications across various industries, including healthcare, automotive, retail, entertainment, and more.
At its core, computer vision seeks to replicate the remarkable ability of humans to extract valuable information from visual inputs effortlessly. This involves tasks such as object recognition, image segmentation, face detection, and even more complex activities like scene understanding and image captioning. Achieving these tasks requires computers to learn from data, making machine learning an integral part of computer vision.
Deep learning, specifically convolutional neural networks (CNNs), has revolutionized the field of computer vision in recent years. CNNs have demonstrated exceptional performance in various computer vision tasks, surpassing human-level accuracy in some cases. They are capable of automatically learning and extracting hierarchical features from images, making them well-suited for tasks like image classification and object detection.
Enhancing Data Analysis
Enhancing data analysis is a critical aspect of extracting valuable insights and making informed decisions in today's data-driven world. It refers to the process of improving the quality, accuracy, and depth of analysis performed on datasets to derive more meaningful and actionable conclusions. This topic encompasses various techniques, methodologies, and best practices aimed at maximizing the potential of data analysis.
One key aspect of enhancing data analysis is data preprocessing. This involves cleaning and transforming raw data to remove inconsistencies, missing values, and outliers. By ensuring data quality, analysts can reduce the chances of drawing incorrect conclusions and enhance the reliability of their findings.
Another essential element of enhancing data analysis is feature engineering, which involves selecting, creating, or modifying the input variables used in analysis. Thoughtful feature selection and engineering can significantly impact the model's performance and the insights derived from it.
In addition to preprocessing and feature engineering, advanced analytics techniques such as machine learning and artificial intelligence play a crucial role in enhancing data analysis. These methods enable the discovery of complex patterns, predictions, and classifications in the data, providing valuable insights that may not be apparent through traditional statistical analysis.
Visualization is another essential tool in the arsenal of data analysts. It helps in representing data in a comprehensible manner, making it easier for stakeholders to grasp trends, relationships, and anomalies. Effective visualization can enhance data communication and facilitate decision-making.
Improved Decision-Making
Advanced Analytics Tools: Utilize advanced analytics tools and software like Python, R, or specialized platforms (e.g., Tableau, Power BI) to improve data analysis capabilities.
Data Visualization: Create meaningful and informative data visualizations (charts, graphs, dashboards) to better understand patterns and trends in the data.
Machine Learning: Incorporate machine learning techniques to uncover insights and make predictions based on data patterns.
Data Preprocessing: Pay attention to data cleaning, normalization, and transformation to ensure data quality before analysis.
Big Data Technologies: Learn and leverage big data technologies like Hadoop, Spark, and NoSQL databases for handling and analyzing large datasets.
Data Mining: Apply data mining techniques to discover hidden patterns, associations, and anomalies in your data.
Domain Knowledge: Combine data analysis with domain expertise to gain deeper insights and make more informed decisions.
Data Governance: Implement data governance practices to maintain data quality, integrity, and security throughout the analysis process.
Data Storytelling: Develop the ability to communicate findings effectively through data storytelling, making insights more accessible to non-technical stakeholders.
Automation and Efficiency
Automation and efficiency are two closely interconnected concepts that have transformed various aspects of modern life, from industries and businesses to everyday tasks. Automation refers to the use of technology and machinery to perform tasks and processes with minimal human intervention. This can include tasks like manufacturing, data entry, and even customer service through the use of chatbots. The primary goal of automation is to streamline operations, reduce errors, and save time and resources.
Efficiency, on the other hand, is the measure of how effectively resources are used to achieve a particular goal or outcome. When automation is employed effectively, it often leads to increased efficiency. By automating repetitive and time-consuming tasks, businesses can allocate their human resources to more creative and strategic roles, which can lead to improved productivity and innovation.
In the business world, automation can be seen in various forms, such as robotic process automation (RPA), which involves software robots performing routine tasks, and the use of artificial intelligence (AI) to make data-driven decisions. These technologies have the potential to revolutionize industries by reducing costs, increasing accuracy, and enhancing the overall quality of products and services.
Customer Experience Enhancement
Customer Experience Enhancement refers to the strategies and initiatives that businesses and organizations undertake to improve and optimize the overall experience their customers have when interacting with their products, services, and brands. In today's highly competitive marketplace, providing an exceptional customer experience has become a crucial element for success. It goes beyond simply selling a product or service; it's about creating a positive and memorable journey for customers at every touchpoint.
One key aspect of customer experience enhancement is understanding and meeting customer expectations. This involves gathering feedback, conducting surveys, and analyzing customer data to gain insights into what customers want and need. By aligning products, services, and processes with these insights, companies can tailor their offerings to exceed customer expectations.
Personalization is another critical component of enhancing customer experiences. Companies are increasingly using data-driven approaches to customize interactions, providing customers with tailored recommendations, content, and solutions. This not only increases customer satisfaction but also boosts loyalty and encourages repeat business.
Effective communication and engagement are also essential. Businesses must maintain open channels of communication with their customers, whether through social media, email, chat, or other means. Providing timely and relevant information, addressing queries promptly, and actively listening to customer feedback can significantly enhance the overall experience.
Challenges and Considerations
Challenges and considerations are essential elements to contemplate when navigating various aspects of life, work, or decision-making. These factors often arise in diverse contexts, such as business, technology, personal relationships, and societal issues. Identifying and addressing challenges and considerations is crucial for making informed choices and achieving success.
In the realm of business, challenges can encompass market competition, economic fluctuations, and rapidly evolving technology. Considerations may involve financial planning, market research, and risk management. Navigating these challenges and considerations is vital for a company's sustainability and growth.
In the realm of technology, innovation brings both opportunities and complexities. Challenges like cybersecurity threats and ethical dilemmas must be addressed alongside considerations related to user privacy, data governance, and compliance with regulations. Striking the right balance between progress and responsibility is key.
In personal relationships, challenges often revolve around communication, trust, and differing values or expectations. Considering factors like empathy, compromise, and open dialogue can help resolve conflicts and strengthen bonds.
On a broader societal scale, challenges like climate change, inequality, and global health crises require collective action and thoughtful considerations about policy, resource allocation, and ethical implications. These issues demand cooperation and long-term thinking to find sustainable solutions.
the complexities of the digital age, the synergy between Computer Vision and Business Intelligence opens up new frontiers of innovation and strategic decision-making. The ability to extract valuable insights from visual data not only propels businesses ahead of the curve but also transforms industries by creating more efficient, customer-centric, and data-driven ecosystems. The future of Business Intelligence is undoubtedly intertwined with the vision of machines, and the organizations that embrace this evolution are poised for a competitive advantage in the ever-evolving business landscape.