Exploring the Real-world Impact of Artificial Intelligence in Data Science
"Discover the tangible effects of Artificial Intelligence in Data Science. Explore real-world applications and insights. Gain a deeper understanding of AI's influence.
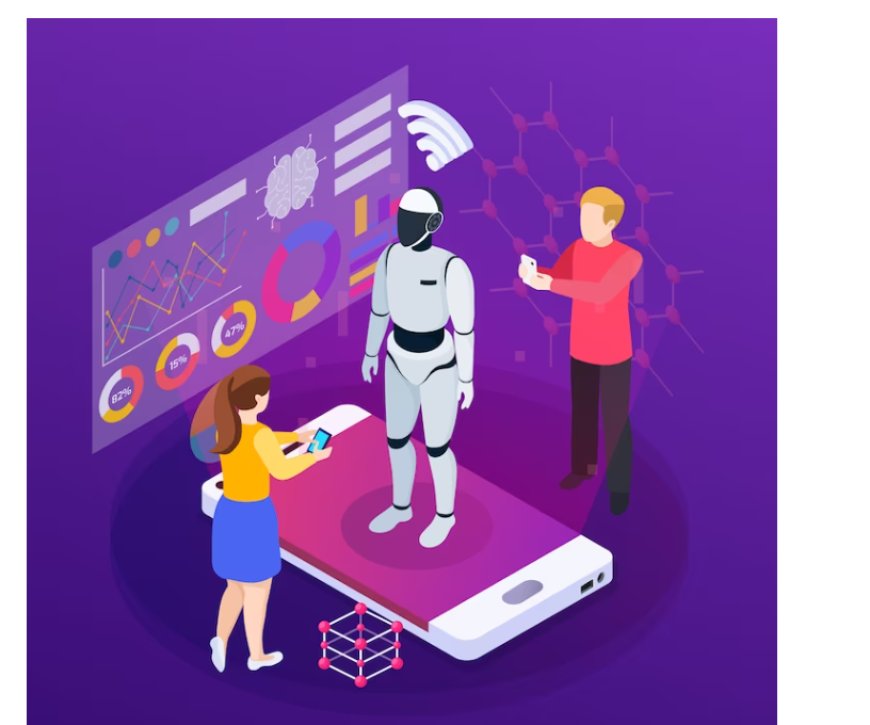
In the ever-evolving landscape of technology, Artificial Intelligence (AI) has emerged as a transformative force, particularly in the field of Data Science. The synergy between AI and Data Science has opened up new possibilities, revolutionizing the way businesses operate and decision-makers leverage insights.
Enhancing Predictive Analytics
Predictive analytics is the process of using historical data, statistical algorithms, and machine learning techniques to identify patterns and trends that can be used to make future predictions. Artificial Intelligence (AI) has significantly enhanced the capabilities of predictive analytics in several ways.
Traditionally, predictive analytics relied on statistical models that often struggled to handle vast and complex datasets. AI, particularly machine learning, has revolutionized this field by offering algorithms that can efficiently process and analyze large volumes of data. These AI models excel at recognizing intricate patterns and relationships within the data that may be challenging for traditional statistical methods to capture.
Machine learning algorithms, such as decision trees, random forests, and neural networks, have the capacity to adapt and learn from data over time. This adaptability enables predictive models to improve their accuracy as they receive more information, making them particularly valuable in dynamic environments where data evolves continuously.
Moreover, AI-driven predictive analytics is not limited to linear relationships between variables. It can uncover complex, nonlinear correlations that might be hidden to human analysts, thus providing more accurate and nuanced predictions. This is particularly advantageous in fields like finance, where predicting stock prices, or in healthcare, where forecasting disease outbreaks, requires a deep understanding of intricate patterns.
Automation of Data Processing
Automation of data processing is a critical aspect of modern data management and analysis. It involves the use of technology, particularly Artificial Intelligence (AI) and machine learning, to streamline and expedite various tasks associated with handling and analyzing large volumes of data. This automation significantly improves efficiency, reduces human errors, and allows data scientists and analysts to focus on more strategic and complex aspects of their work. Here are some key points to understand about the automation of data processing
Data Collection and Ingestion: Automation in data processing starts with the collection and ingestion of data from various sources. Automated tools can gather data from databases, APIs, websites, IoT devices, and other sources, ensuring a continuous flow of data into the data processing pipeline.
Data Cleaning: Raw data is often messy, containing missing values, duplicates, inconsistencies, and errors. Data cleaning involves automating the process of identifying and rectifying these issues. AI algorithms can detect anomalies and perform data imputation to ensure that the data is accurate and complete.
Feature Engineering: Feature engineering is a crucial step in preparing data for analysis. It involves transforming raw data into a format that is suitable for machine learning algorithms. Automation can assist in identifying relevant features, creating new ones, and scaling or normalizing data as needed.
Data Transformation: Data often needs to be transformed to meet the requirements of specific analyses or models. Automation tools can perform operations such as data pivoting, aggregations, and calculations efficiently and consistently.
Data Integration: In many cases, data processing involves integrating data from multiple sources. Automation can handle data integration tasks, ensuring that data from different sources is harmonized and ready for analysis.
Unleashing the Power of Natural Language Processing (NLP)
Natural Language Processing (NLP) is a fascinating branch of Artificial Intelligence that focuses on the interaction between humans and computers through natural language. In essence, it allows computers to understand, interpret, and generate human language. This technology has seen significant advancements in recent years, and its real-world impact is profound.
One of the key applications of NLP is in text analysis and comprehension. NLP algorithms can process vast amounts of textual data, ranging from news articles and social media posts to customer reviews and emails. They can extract valuable insights from this unstructured text, enabling businesses to gain a deeper understanding of their customers, market trends, and competitor strategies.
Sentiment analysis is another powerful use case of NLP. By analyzing the sentiment expressed in customer reviews or social media comments, businesses can gauge public opinion about their products or services. This information is invaluable for reputation management, product improvement, and marketing strategy adjustments.
Personalizing User Experiences
In today's digital age, personalization has become a cornerstone of effective user engagement, and it is here that the influence of Artificial Intelligence in Data Science is particularly pronounced. Personalizing user experiences entails tailoring interactions, content, and recommendations to the individual preferences, behaviors, and needs of each user. AI, through machine learning and predictive analytics, plays a pivotal role in achieving this level of customization.
One of the most notable examples of personalization is seen in the realm of e-commerce. AI-powered recommendation engines analyze vast amounts of data, including past purchase history, browsing behavior, and demographic information, to suggest products and services that are highly relevant to each user. This not only enhances the user's experience by simplifying the discovery process but also drives higher conversion rates and increased sales for businesses.
In the content industry, personalization is equally impactful. Streaming services like Netflix and music platforms like Spotify leverage AI algorithms to curate playlists, movies, and TV shows tailored to the viewer's preferences. These platforms analyze data on what users watch, listen to, and rate, creating a personalized content catalog that keeps users engaged and satisfied.
Addressing Data Security Challenges
Data Encryption: Implementing robust encryption techniques to protect data both at rest and in transit, ensuring that even if unauthorized access occurs, the data remains unreadable.
Access Control: Implementing stringent access control measures to limit who can view, modify, or delete sensitive data, and ensuring that permissions are regularly reviewed and updated.
Data Masking/Anonymization: Utilizing techniques like data masking or anonymization to hide or replace sensitive information with non-sensitive data, allowing for safe data sharing and analysis.
Regular Auditing and Monitoring: Setting up systems for continuous monitoring and auditing of data access and usage to detect any suspicious or unauthorized activities promptly.
Secure APIs: Ensuring that Application Programming Interfaces (APIs) used for data access are secured with proper authentication and authorization mechanisms.
Data Classification: Classifying data based on its sensitivity and implementing different security measures accordingly, such as stronger encryption for highly sensitive data.
User Training and Awareness: Conduct regular training and awareness programs to educate employees about data security best practices and the importance of safeguarding data.
Incident Response Plan: Develop a well-defined incident response plan to handle data breaches, including steps for containment, investigation, and communication with affected parties.
Overcoming Bias and Ethical Concerns
The integration of Artificial Intelligence (AI) into Data Science brings with it the challenge of overcoming biases and addressing ethical concerns. AI models, trained on historical data, can inadvertently perpetuate existing biases present in the datasets. These biases may stem from societal prejudices, systemic inequalities, or flawed human decision-making processes reflected in the data. Consequently, there is a pressing need for ethical considerations in the development and deployment of AI applications.
One key aspect of overcoming bias involves raising awareness within the AI community and beyond about the potential pitfalls associated with biased algorithms. Researchers and developers must be vigilant in identifying and rectifying biased outcomes, as well as transparently communicating the limitations of their models. The responsibility to create fair and unbiased AI lies not only with the developers but also with the organizations and policymakers who deploy these technologies.
Ethical concerns extend beyond mere technical issues, encompassing broader societal implications. As AI systems increasingly influence decision-making in areas such as hiring, lending, and criminal justice, the need for fairness and accountability becomes paramount. Strides are being made towards developing frameworks and guidelines that promote ethical AI, encouraging the development of models that are transparent, explainable, and free from discriminatory practices.
Democratizing Data Science
Democratizing Data Science is a concept that aims to make the field of data analysis and data-driven decision-making accessible to a broader audience, beyond traditional data scientists and experts. It involves the development and adoption of tools, platforms, and methodologies that empower individuals with diverse backgrounds, skills, and roles to engage in data analysis and derive meaningful insights from data. This democratization of data science has become increasingly important as organizations recognize the value of data-driven decision-making in today's data-rich environment.
Accessibility for Non-Experts: Democratizing data science means breaking down the barriers to entry and creating user-friendly interfaces and tools that allow individuals who may not have a background in statistics or programming to work with data. This can include business analysts, marketers, product managers, and other professionals who can benefit from data insights in their roles.
User-Friendly Data Tools: The development of user-friendly data analysis tools and platforms with intuitive interfaces is a fundamental aspect of democratizing data science. These tools often feature drag-and-drop functionalities, pre-built templates, and automated analysis options, reducing the need for extensive coding or technical expertise.
Self-Service Analytics: Democratization also involves enabling self-service analytics, where end-users can access, explore, and analyze data on their own without relying on dedicated data science or IT teams. Self-service analytics platforms provide user-friendly dashboards and visualization tools that make data exploration and reporting accessible to non-technical users.
Education and Training: Democratizing data science requires providing accessible and affordable training and education opportunities. Online courses, tutorials, and resources make it possible for individuals to acquire the basic skills needed to work with data and analytical tools, regardless of their location or background.
Collaboration and Knowledge Sharing: Collaboration tools and platforms that facilitate knowledge sharing and collaboration among teams are essential. These platforms allow experts and non-experts to work together, share insights, and collectively make data-informed decisions.
The real-world impact of Artificial Intelligence in Data Science is multifaceted, bringing about positive transformations in various industries. From predictive analytics to personalized user experiences, the integration of AI is reshaping the way businesses operate and make decisions. However, this transformative journey is not without challenges, and addressing issues related to data security, bias, and ethics is crucial for realizing the full potential of AI in the realm of Data Science.