The Synergy of Machine Learning and Social Robotics
Explore the fascinating synergy between machine learning and social robotics. Discover how AI-driven advancements are reshaping human-robot interactions and applications.
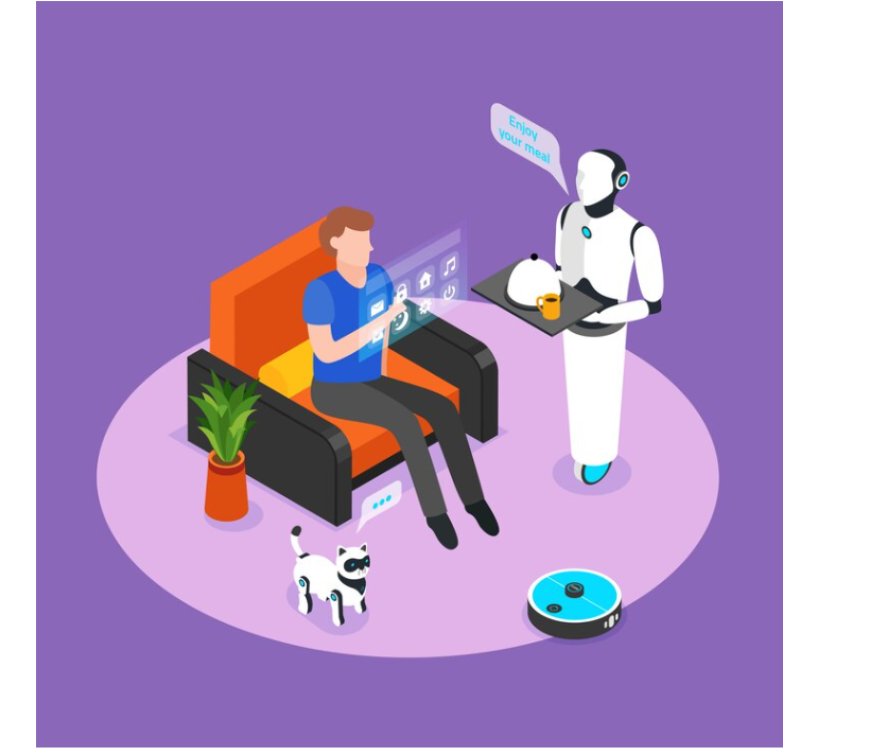
In the realm of technological innovation, the convergence of machine learning and social robotics stands as a testament to the boundless possibilities that emerge when cutting-edge technologies collaborate. Social robotics, the field dedicated to creating machines capable of interacting with humans in a socially intelligent manner, has witnessed a remarkable transformation owing to the integration of machine learning algorithms.
Enhanced Human-Robot Interaction (HRI)
Enhanced Human-Robot Interaction (HRI) is a transformative aspect of social robotics that has been significantly advanced through the integration of machine learning. In the past, human-robot interactions often felt stiff and scripted, lacking the spontaneity and adaptability that characterize natural human communication. However, with the infusion of machine learning algorithms, social robots have made significant strides in improving the quality of these interactions.
One of the key contributions of machine learning to HRI is its ability to enable robots to learn from data and adapt to the ever-changing dynamics of human behavior. Traditional rule-based approaches struggled to account for the vast spectrum of human communication styles and preferences. Machine learning algorithms, particularly those associated with natural language processing (NLP), have allowed robots to comprehend and respond to human speech with greater contextual understanding.
For example, social robots equipped with machine learning can recognize speech patterns, understand context, and even detect emotions in the tone of voice. This means that in a conversation, a robot can gauge if a person is happy, sad, or frustrated and adjust its responses accordingly. This level of responsiveness enhances the authenticity of the interaction, making it feel less like an interaction with a machine and more like a conversation with a sentient being.
Ethical and Social Implications
The integration of machine learning into social robotics brings forth a host of ethical and social implications that need careful consideration. These implications touch on various aspects of society and human interaction with advanced robots. Here are some key points to explore in this section:
- Privacy Concerns: Social robots equipped with machine learning algorithms may collect and process vast amounts of personal data to improve their interactions with users. This raises questions about the privacy of individuals who interact with these robots. How is this data stored and secured? Who has access to it, and for what purposes? Striking a balance between customization and respecting user privacy is a significant ethical challenge.
- Bias and Fairness: Machine learning models used in social robotics can inadvertently inherit biases present in their training data. If not carefully curated, these biases can lead to unfair or discriminatory behavior by social robots. Ethical considerations involve ensuring that algorithms are trained on diverse and unbiased datasets to avoid perpetuating harmful stereotypes or prejudices.
- Social Impact: The widespread adoption of social robots, particularly in roles that involve human-like interactions, could have significant societal repercussions. For example, there is a concern that increased reliance on social robots might lead to job displacement, particularly in fields like customer service. Addressing the potential economic and employment consequences of automation is a crucial aspect of ethical decision-making.
- Psychological and Emotional Implications: Interactions with social robots can elicit emotional responses from humans. People may develop emotional bonds with robots, leading to questions about the ethical implications of these emotional attachments. How should society address the potential for loneliness or dependency on robots for emotional support?
Personalized Experiences
Personalization is a key aspect of how machine learning is advancing social robotics. In the context of social robots, personalized experiences refer to tailoring the interactions and responses of these machines to suit the individual needs, preferences, and behavioral patterns of their users. This concept is integral to making human-robot interactions more meaningful and engaging.
One of the most significant advantages of personalized experiences in social robotics is the ability to create a deeper connection between the user and the robot. By analyzing user data and feedback, social robots can adapt their behavior to align with the specific requirements of each user. For example, a healthcare robot interacting with patients suffering from various medical conditions can provide personalized reminders for medication, exercises, or dietary restrictions, thereby improving patient adherence and overall well-being.
Personalized experiences also enhance the efficiency and effectiveness of social robots in various domains. In educational settings, for instance, a robot tutor can assess the learning pace and preferences of individual students. It can then customize its teaching methods and materials, ensuring that each student receives targeted support and a more effective learning experience. This not only improves learning outcomes but also boosts students' motivation and engagement.
Cognitive Learning and Problem-Solving
Cognitive learning and problem-solving are essential aspects of how machine learning is advancing social robotics. These capabilities enable social robots to go beyond basic interactions and perform more complex tasks, making them valuable assets in various fields. Here's a closer look at cognitive learning and problem-solving in the context of social robotics:
- Continuous Learning: Social robots equipped with cognitive learning capabilities have the ability to acquire new knowledge and skills over time. Unlike traditional robots with fixed programming, these robots can adapt to changing environments and tasks. This adaptability is crucial for applications such as household assistance, where the robot needs to learn and adapt to various household chores.
-
Adaptive Behavior: Cognitive learning allows social robots to modify their behavior based on the information they acquire. For example, a robot assisting in a classroom setting can adjust its teaching style to match the learning pace and preferences of individual students, enhancing the educational experience.
-
Problem-solving skills: Social robots with problem-solving capabilities can analyze complex situations and make decisions to achieve specific goals. This is particularly valuable in scenarios where robots need to assist in troubleshooting technical issues, managing resources efficiently, or providing assistance during emergencies.
-
Dynamic Task Allocation: In environments where multiple robots collaborate, cognitive learning and problem-solving come into play when allocating tasks efficiently. Robots can assess the urgency, complexity, and their own capabilities to determine which tasks they should undertake. This dynamic task allocation enhances overall teamwork and productivity.
-
Adapting to Novel Situations: Cognitive learning enables social robots to handle novel situations or tasks that were not explicitly programmed. These robots can leverage their knowledge base and problem-solving skills to respond to unexpected challenges, making them more versatile and reliable in various applications.
Social Learning and Collaboration
One of the groundbreaking advancements catalyzed by the marriage of machine learning and social robotics is the concept of social learning and collaboration among robotic entities. In essence, social robots are no longer solitary entities bound by their individual experiences; instead, they form a collective network capable of shared learning and knowledge exchange.
In practical terms, this means that a social robot can glean insights not only from its own interactions with humans but also from the experiences of other robots within its network. Imagine a scenario where one robot encounters a novel situation and successfully navigates it; this information can be transmitted to other robots in the network. Through machine learning algorithms, these robots can assimilate and adapt the knowledge gained collectively, creating a dynamic and evolving pool of expertise.
This collaborative learning approach is particularly advantageous in environments where multiple social robots operate together, such as in large-scale service industries or educational settings. For instance, in a healthcare facility where robots assist patients, a robot encountering a unique medical scenario can share its observations and solutions with other robots. This collective learning not only enhances the overall competency of the robotic team but also enables them to tackle a diverse range of challenges more effectively.
The fusion of machine learning and social robotics has unlocked a new era of possibilities, pushing the boundaries of what robots can achieve in the realm of human interaction. From healthcare and education to customer service and daily companionship, social robots are becoming integral parts of our lives. As these machines continue to evolve, driven by advancements in machine learning, the future holds the promise of even more sophisticated and socially intelligent robots that seamlessly integrate into our daily routines, offering unprecedented levels of assistance, companionship, and enrichment.