Data Science Finance Techniques for Investing
Learn powerful finance techniques using data analysis, predictive modeling, and machine learning to make smarter investment decisions. Simplified guide for better financial choices.
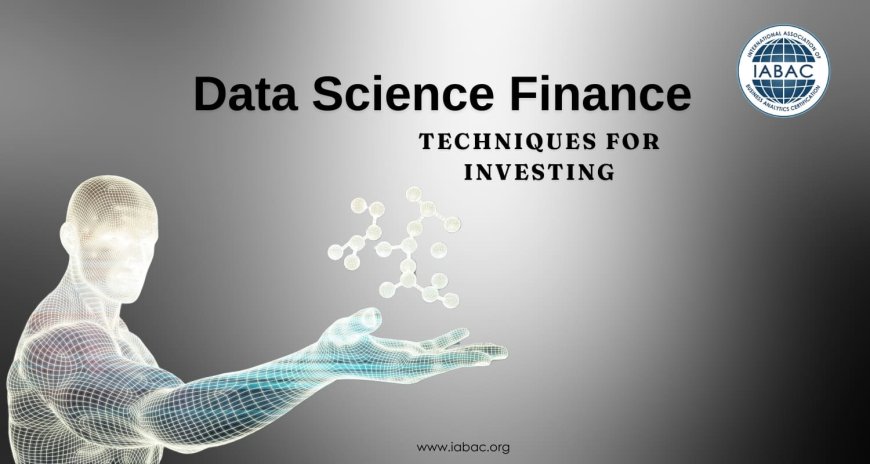
Using data science techniques is important for making good investment choices. Data science finance combines methods like getting data, using machines to learn from it, and showing it visually. These methods help investors understand lots of data and make smart decisions about where to invest. Getting the right data is key in data science finance. It means gathering different kinds of information from markets and even social media. Machine learning helps by looking at past data and predicting what might happen in the future. Deep learning goes deeper, finding complex patterns in the data.
Visualizing data is also important. It makes complicated financial information easy to understand quickly. With data science tools, investors can manipulate data easily and make decisions based on accurate insights. The finance industry is using big data and analytics more and more. Responsible AI in data science finance is about making sure algorithms are fair and ethical, without biases that could harm anyone.
Mastering Investments with Data Science Finance
In finance, decisions can make or break fortunes. Data science finance is changing how we invest money. It combines traditional financial analysis with fancy data techniques. This helps investors understand the tricky market better. Data collection is the first step in data science finance. We gather lots of financial data from different places. Then, we use smart computer programs to study this data. These programs help us find important information. With this info, investors can make smart decisions using predictions instead of just guessing.
Making sense of all this data is hard. But, data visualization makes it easier. It helps investors see trends and patterns in the numbers. We use special tools to help with this. Using AI responsibly is very important. We want our computer programs to be fair and reliable. When AI is used responsibly, investors can trust their strategies more. Data science and finance together are changing how we invest. They give investors the tools they need to understand the market better. By using data collection, smart programs, visualization, and responsible AI, data science finance helps us make better decisions with our money.
The Complications of Data Science Finance Techniques for Investing
Using data science techniques to make investment decisions has become more common. These techniques offer investors helpful tools, but they also come with some challenges. Let's look at some of the main issues with using data science in finance:
1. Quality of Data and Bias: Making good decisions in data science finance relies on having accurate data. Financial data can be big and varied, and sometimes it's not consistent. Also, there might be biases in how the data is collected or understood, which can make investment decisions go wrong.
2. Complex Models vs. Easy Understanding: Fancy machine learning models can be good at predicting what will happen in the market. But sometimes they're so complicated that it's hard for people to understand them. This can make it tough for investors to trust what the models are saying.
3. Fitting Too Closely to Past Data: Models in data science finance can be great at predicting what happened in the past. But they might not be so good at guessing what will happen in the future. Sometimes they focus too much on tiny details from the past and don't see the bigger picture.
4. Markets Always Changing: Financial markets are always changing because of things like the economy, politics, and new technology. Models in data science finance might not be able to keep up with these changes, which can make their predictions wrong.
5. Following Rules and Being Ethical: Using data science in finance means following rules about how data is used and being ethical about it. Investors have to make sure they're following the law and being fair when they use data science techniques. They also have to watch out for biases and unintended consequences.
6. Keeping Data Safe: More and more financial stuff is happening online, which means there's a bigger risk of someone hacking into it. If someone gets into the data, they can mess up investment plans that rely on data science.
7. Using Both Machines and People: Data science tools are great, but people still need to make decisions too. It's important to find the right balance between using machines and using human judgment. Relying too much on machines can lead to bad decisions.
How can Data Science Finance techniques improve investment strategies?
Data Science Finance techniques can change how investments are made by using smart computer programs and math to find patterns in big sets of data. This helps investors make better decisions based on facts and predictions, making it easier to spot trends and foresee market changes. By using fancy math like regression analysis and neural networks, investors can better understand how markets work and reduce risks. Also, they can build diversified investment plans using methods like factor modeling and portfolio optimization, which means they can balance risks and rewards more effectively. By looking at unusual data like social media feelings, satellite pictures, and different financial clues, investors can find good chances and predict market moves before others do. Data Science Finance helps investors handle unpredictable markets better and make more money.
Understanding Data Science in Finance for Investing
Financial world, investors are using data science techniques to make smarter investment decisions. Here are some simple ways to do it:
1. Number Crunching: This means using math and stats to look at financial data. By studying past patterns, investors can figure out the risks and rewards of different investments. This helps them create better strategies for buying and selling.
2. Smart Computers: Machine learning helps computers analyze lots of data and make predictions. In finance, these predictions can be used to guess stock prices, manage risks, and spot fraud. Techniques like decision trees and neural networks are used to find valuable insights in financial data.
3. Feeling the Market: Sentiment analysis looks at things like news articles and social media posts to see how people feel about stocks. By understanding these feelings, investors can spot trends and predict what the market might do next. Natural language processing helps turn written words into useful insights.
4. Building the Best Portfolio: Portfolio optimization is about creating a mix of investments that gives the best returns with the least risk. Data science helps investors figure out the perfect combination of assets. By considering things like how different investments are connected and how likely they are to go up or down in value, investors can build a portfolio that matches their goals.
5. Looking at Unusual Data: Alternative data is info that's not usually used in finance, like satellite images or credit card transactions. By analyzing this kind of data, investors can learn things about companies and industries that others might miss. Data science helps investors find the important bits in all this extra info and use it to make better decisions.
Data Science Finance: Smart Techniques for Investing
Data science has changed the way people invest in finance by using smart techniques:
1. Predictive Analysis: This is like predicting the future. Computers use past data to guess what might happen in the market.
2. Sentiment Analysis: This checks how people feel about the market by looking at news and social media. It helps predict if prices will go up or down.
3. Portfolio Optimization: This is about making your investments work best for you. Computers use math to figure out the best way to invest your money for the most profit with the least risk.
4. Algorithmic Trading: This means letting a computer automatically make trades for you. It uses specific rules based on what's happening in the market right now.
5. Time Series Analysis: This looks at how things change over time, like stock prices or economic indicators. It helps predict what might happen next.
6. Alternative Data Integration: This means using different kinds of data, like pictures from satellites or weather information, to get even more insights.
7. Risk Management with Monte Carlo Simulations: This helps you understand how risky your investments are. It uses computer simulations based on past data to see what might happen in different situations.
Mixing data science with finance is making investing smarter. It brings in new ways to understand and grab opportunities for investors. With tools like machine learning and predictive analytics, everyone from individuals to big companies can make better choices, manage their investments well, and lower risks. These smart methods help investors deal with the complicated world of finance more accurately and quickly. As technology keeps getting better, the teamwork between data science and finance will keep changing how people invest. It will lead to a future where using data is key to financial success.