Data Science for Social Good: Making an Impact with Analytics
Explore the transformative power of data science for social good. Learn how analytics can make a positive impact on society, addressing critical issues and driving meaningful change.
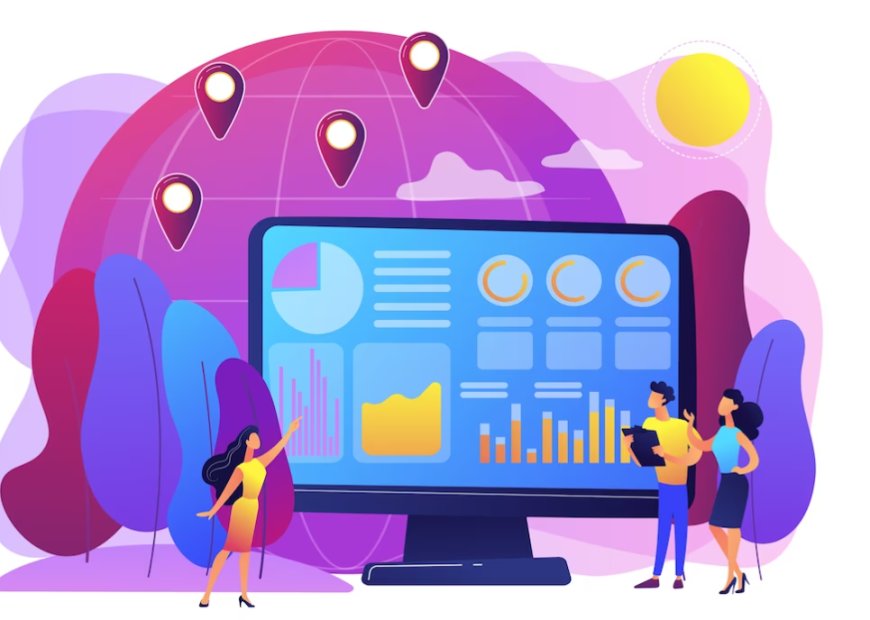
In today's data-driven world, the power of data science extends far beyond the business realm. Data science has the potential to drive positive change and make a significant impact on society. This emerging field, often referred to as "Data Science for Social Good," leverages the tools and techniques of data analytics to address complex social challenges, improve public services, and advance the well-being of communities.
What is Data Science for Social Good?
Data Science for Social Good (DSSG) is a dynamic and purpose-driven field that employs the principles and methodologies of data science to tackle pressing social and humanitarian challenges. At its core, DSSG seeks to leverage the analytical capabilities of data scientists to generate meaningful insights and solutions that can address complex issues affecting society.
Unlike traditional data science applications primarily focused on business and industry, DSSG is characterized by a strong emphasis on social impact. This interdisciplinary approach involves collaboration between data scientists, domain experts, nonprofits, government agencies, and other stakeholders. The overarching goal is to harness the power of data analytics to inform decision-making, shape evidence-based policies, and drive positive change in areas such as healthcare, education, poverty alleviation, and disaster response.
Ethical considerations play a pivotal role in DSSG, ensuring that data-driven solutions are not only effective but also respectful of privacy, unbiased, and transparent. The ultimate measure of success in DSSG lies in its ability to make a tangible and positive difference in the lives of individuals and communities, marking a departure from the conventional applications of data science towards a more socially conscious and impactful paradigm.
Key Principles of Data Science for Social Good
-
Collaborative Approach
DSSG projects often involve collaboration between data scientists, nonprofit organizations, government agencies, and other stakeholders. This multidisciplinary approach ensures that the insights and solutions generated are relevant and actionable within the specific context of the problem.
-
Ethical Considerations
Ethical considerations are paramount in DSSG. Data scientists working on social good projects must adhere to strict ethical guidelines to ensure privacy, fairness, and transparency in their work. Protecting sensitive information and addressing bias are critical aspects of this.
-
Impact-Driven
The ultimate measure of success in DSSG is the impact on society. Projects are designed with clear goals and metrics to assess their effectiveness in solving the identified social problem. This impact-oriented approach distinguishes DSSG from purely academic or commercial data science projects.
Examples of Data Science for Social Good
-
Predictive Policing
Predictive policing is an application of data science that aims to make law enforcement more proactive and effective. By analyzing historical crime data, social demographics, and environmental factors, predictive policing models can identify areas at higher risk of criminal activity. This information allows police departments to allocate their resources strategically, focusing on potential hotspots and thereby reducing crime rates. However, it's crucial to handle this technology with care to ensure it doesn't lead to biased or discriminatory policing practices.
-
Healthcare and Epidemic Control
The COVID-19 pandemic highlighted the vital role of data science in healthcare and epidemic control. Data-driven models tracked the spread of the virus, predicted hotspots, and informed decisions on lockdowns and resource allocation. This approach saved lives and reduced the strain on healthcare systems. Additionally, vaccine distribution strategies were optimized using data analysis to ensure equitable access to vaccines across different populations, contributing to the global fight against the pandemic.
-
Disaster Response
Data science is instrumental in disaster response efforts. When natural disasters strike, such as hurricanes, earthquakes, or wildfires, predictive models can estimate the impact and trajectory of these events. This information enables emergency responders to prepare adequately, evacuate affected areas, and allocate resources where they are most needed. Data-driven insights improve the efficiency and effectiveness of disaster response, ultimately saving lives and minimizing damage.
-
Poverty Alleviation
Data analytics plays a crucial role in addressing poverty and inequality. By analyzing socioeconomic data, governments and organizations can identify trends and patterns that inform policies and interventions aimed at alleviating poverty. These efforts can lead to targeted investments in education, healthcare, and social services, creating opportunities for marginalized communities to improve their quality of life and break the cycle of poverty.
Challenges in Data Science for Social Good
Data Science for Social Good (DSSG) holds tremendous promise in addressing pressing societal issues, but it is not without its challenges. One significant hurdle lies in the realm of data access and quality. In many cases, particularly in underserved or marginalized communities, access to high-quality data is limited. This challenge hampers the ability of data scientists to derive accurate insights and build effective models. Ensuring data accuracy and relevance becomes crucial in navigating this constraint, often requiring innovative approaches to gather and verify information.
Ethical concerns are another pivotal challenge in DSSG. The responsible use of data is paramount, and considerations such as privacy, bias, and potential harm require ongoing attention. As DSSG projects often involve vulnerable populations or sensitive information, data scientists must navigate complex ethical landscapes to ensure that their work does not inadvertently contribute to social inequalities or compromise individuals' privacy.
Resource constraints pose a third challenge in the field. Many social organizations lack the necessary resources and expertise to fully leverage the potential of data science. Bridging this gap often necessitates collaboration and support from the broader data science community. Efforts to democratize access to data science tools, knowledge, and resources can play a crucial role in empowering organizations and communities to harness the benefits of data-driven solutions.
How You Can Get Involved
Getting involved in Data Science for Social Good (DSSG) is not just a theoretical proposition; it's a call to action for individuals who want to leverage their skills for positive change. There are various avenues through which you can contribute to this impactful movement.
-
Volunteering: One of the most direct ways to make a difference is by volunteering your data science skills. Many nonprofits and community organizations actively seek individuals with expertise in data analytics. Whether it's helping a local organization analyze community health data or assisting in optimizing resource allocation for a charitable cause, your skills can be a valuable asset.
-
DSSG Programs and Internships: Numerous universities and institutions offer DSSG programs and internships that provide hands-on experience in tackling real-world social challenges. Participating in these programs not only allows you to apply your skills in a meaningful way but also exposes you to the collaborative and interdisciplinary nature of DSSG.
-
Collaboration: Reach out to organizations and agencies that align with your values and interests. Many social organizations may lack the expertise in data science but could greatly benefit from its application. By initiating collaboration, you can offer your skills to develop data-driven solutions for issues such as poverty, education, or healthcare.
-
Advocacy for Ethical Data Science: Advocacy is a powerful way to contribute to the field. Promote the importance of ethical data science practices within your professional community. This includes emphasizing privacy, fairness, and transparency in all data-related activities. By advocating for responsible data use, you contribute to building a sustainable and ethical foundation for DSSG.
In essence, your involvement in DSSG is not limited to your technical expertise alone. It extends to your willingness to collaborate, your passion for positive change, and your advocacy for ethical practices. By taking these steps, you become an integral part of the movement, helping to shape a future where data science is a force for social good.
Data Science for Social Good is more than just a buzzword; it's a powerful movement that is making a tangible impact on society. By applying data-driven insights to address complex social challenges, DSSG is helping to create a better world. Whether you're a data scientist, a domain expert, or simply someone passionate about making a difference, there are opportunities for you to contribute to this important and growing field. Together, we can harness the power of data for the greater good and build a more equitable and sustainable future.