Decoding Data Science Certifications
Explore the various data science certifications available, highlighting their significance, and how they can boost your career in the data-driven world.
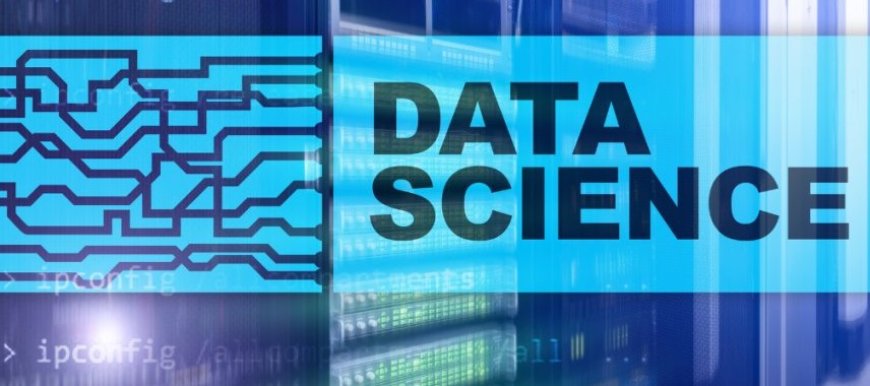
Data Science certifications are credentials awarded to individuals who have demonstrated proficiency and knowledge in the field of data science. These certifications serve as a validation of a person's skills in areas such as statistical analysis, machine learning, data visualization, and programming. They are often offered by reputable organizations, educational institutions, or technology companies. Acquiring a Data Science certification can enhance a professional's credibility in the industry, making them more attractive to employers seeking individuals with expertise in extracting meaningful insights from data
These certifications typically involve a combination of coursework, practical exercises, and exams, ensuring that certified individuals possess a well-rounded understanding of the principles and techniques essential for success in the field of data science. While certifications can provide a competitive edge in the job market, they are often complemented by practical experience and a continuous commitment to learning, as the environment changes with advancements in technology and methodologies.
Difference between entry-level certifications, intermediate certifications, and advanced certifications in data science
-
Entry-level certifications in data science are for beginners, covering basics like statistical analysis and programming. Examples include Microsoft Certified: Data Analyst Associate.
-
Intermediate certifications suit those with some experience, delving into advanced topics like machine learning algorithms and data engineering. Certifications like Cloudera Certified Data Scientist cater to mid-level proficiency.
-
Advanced certifications, such as Certified Data Scientist Expert, are for seasoned professionals, covering complex areas like deep learning and big data analytics. These certifications signify a high level of expertise and are suitable for leadership roles in data science.
Difficulty in choosing a certification that aligns with career goals and personal growth
-
Assess Career Goals: Begin by clearly defining your career goals in the field of data science. Identify the specific areas or roles you aspire to work in, such as data analysis, machine learning, or big data engineering.
-
Research Certification Options: Conduct thorough research on available certifications. Explore reputable organizations and platforms offering certifications in your areas of interest. Consider factors like industry recognition, course content, and practical applicability.
-
Skill Gap Analysis: To Examine your current skill set and identify areas where you need improvement. Choose a certification that addresses your skill gaps and aligns with the skills demanded by your target job roles.
-
Consider Specializations: Data science certifications often come with various specializations. Choose one that aligns with your career goals. For example, if you're interested in natural language processing, look for certifications that offer a specialization in that area.
-
Industry Relevance: Ensure the certification is relevant to the industry or sector you intend to work in. Some certifications may be more valued in specific industries, so align your choice with the industry requirements.
-
Practical Application: Look for certifications that provide hands-on experience or practical projects. Practical application of skills gained through the certification can significantly contribute to your personal growth and readiness for the job market.
-
Networking Opportunities: Some certifications come with access to a community or network of professionals in the field. Consider certifications that offer networking opportunities, as connecting with others can provide insights, mentorship, and potential career growth.
Choosing the right certification that aligns with career goals and personal growth can be challenging. It requires careful consideration of one's professional aspirations and the skills needed to achieve them. Individuals may face a multitude of certification options, each tailored to different aspects of a field, making the decision-making process complex. It's essential to assess both short-term career objectives and long-term growth plans to ensure the selected certification not only meets current job requirements but also contributes to continuous skill development.
How can one identify and prioritize their career goals before selecting a data science certification? What steps can individuals take to assess their current skill set and identify the gaps that certification could fill for their career goals?
To identify and prioritize career goals before selecting a data science certification, individuals should engage in self-reflection, considering personal interests and long-term aspirations within the data science field. They should research industry trends, network with professionals, and analyze job requirements for roles aligned with their goals. Assessing current skills, identifying gaps, and researching certifications that align with industry demands can help individuals make informed choices. Additionally, cost-benefit analysis and consideration of flexible learning options should be part of the decision-making process, ensuring that the selected certification contributes meaningfully to career advancement and personal growth.
-
Self-Evaluation: Reflect on your proficiency in key data science areas, such as programming languages (e.g., Python, R), statistical analysis, and machine learning. Assess your comfort level with relevant tools and technologies.
-
Industry Research: Stay informed about the latest trends and skill requirements in the data science industry. Understand the specific skills demanded by employers in the roles and industries you find appealing.
-
Networking and Mentorship: Connect with professionals working in data science through networking events or online platforms. Seek mentorship to gain valuable insights into the skills that are highly regarded in the field.
-
Job Analysis: Analyze job descriptions for the roles you aspire to, paying close attention to the skills and qualifications listed. This will help you tailor your skill development efforts to meet industry expectations.
-
Skill Inventory: Compile a comprehensive list of your current skills, both technical (coding, data manipulation) and soft skills (communication, problem-solving), relevant to data science.
-
Gap Identification: Compare the skills required for your target roles with the skills in your inventory. Identify gaps or areas where your skills may need enhancement to meet the demands of your desired career path.
-
Project Experience: Gain practical experience by working on data science projects. This hands-on approach not only reinforces theoretical knowledge but also helps identify specific areas where you may lack practical expertise.
-
Feedback Mechanism: Seek feedback from peers, mentors, or professionals who are experienced in data science. Their input can provide valuable perspectives on your strengths and areas for improvement that you might not have considered.
To effectively navigate the data science certifications, one must adopt a strategic and deliberate approach. The journey commences by gaining a clear comprehension of personal career objectives, which necessitates introspection, thorough industry exploration, and networking to pinpoint specific areas of interest. The process then proceeds with a meticulous evaluation of existing skills, identifying gaps that require filling to target desired roles. The significance of researching certification options, considering specializations, and assessing industry relevance cannot be emphasized enough. Moreover, the practical application of skills through practical projects enhances personal growth and prepares individuals for employment. Ultimately, this journey entails a continuous cycle of self-assessment, feedback, and adaptation to ever-changing industry trends. By following these steps, individuals can make well-informed decisions that align with their aspirations, ensuring that chosen certifications contribute significantly to both career progression and ongoing professional development in the dynamic field of data science.