The Power of AI: A Journey into Advanced Data Analysis
Explore the transformative role of AI in advanced data analysis and discover how artificial intelligence is shaping the future of data-driven decision-making.
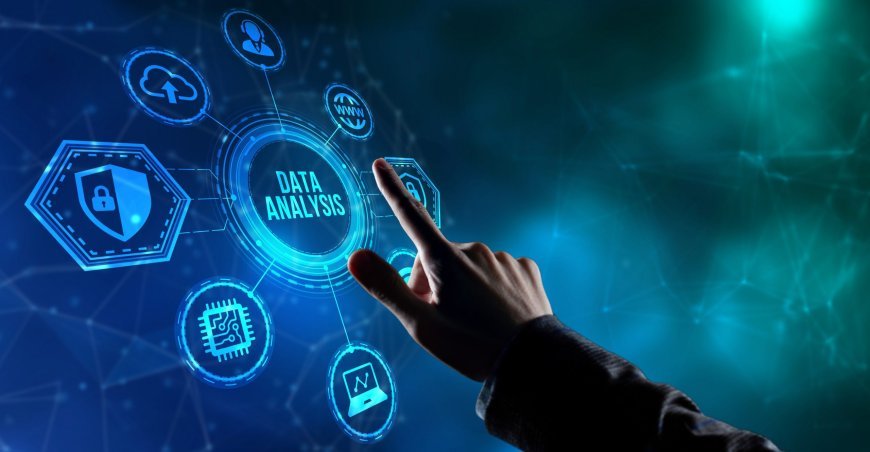
In the era of data abundance, businesses and researchers alike are grappling with the challenge of extracting meaningful insights from vast datasets. As traditional methods struggle to keep pace with the ever-growing volume and complexity of data, Artificial Intelligence (AI) emerges as a transformative force in the realm of advanced data analysis.
The Evolution of Data Analysis
Data analysis has been an essential component of human decision-making and problem-solving for centuries. It began with rudimentary methods like counting, measuring, and simple statistical analysis. However, as societies progressed and the complexity of data increased, so did the methods employed for analysis. The evolution of data analysis can be seen as a continuum that spans from manual calculations to the era of Artificial Intelligence (AI).
In its early stages, data analysis was a labor-intensive process. People relied on pen-and-paper calculations, charts, and graphs to gain insights from data. Statistics, particularly descriptive and inferential statistics, played a crucial role in making sense of data. With the advent of computers in the mid-20th century, data analysis began to take on a new form. Basic statistical software and spreadsheets like Excel facilitated more efficient calculations and data visualization.
The evolution of data analysis took a significant leap with the introduction of specialized software for statistical analysis, such as SPSS and SAS. These tools made it easier to conduct complex statistical tests and generate meaningful reports, but they still required a deep understanding of statistical concepts and a fair amount of manual intervention.
However, as data volumes continued to swell, traditional methods began to show their limitations. The explosion of data in the digital age, commonly referred to as "Big Data," created a pressing need for more powerful and automated data analysis tools. This is where Artificial Intelligence entered the scene.
AI brought about a revolution in data analysis by introducing machine learning algorithms. Machine learning algorithms are capable of learning from data, identifying patterns, and making predictions or decisions without being explicitly programmed. This marked a shift from manual, rule-based analysis to data-driven, algorithmic analysis. Predictive modeling, clustering, classification, and anomaly detection became more accessible, enabling businesses and researchers to extract insights from data at an unprecedented scale and speed.
Machine Learning: The Engine Driving Advanced Data Analysis
Machine Learning (ML) serves as the engine driving advanced data analysis, revolutionizing the way data is processed, interpreted, and leveraged for decision-making. Here's a more detailed explanation of this crucial aspect of data analysis:
Predictive Modeling: Machine Learning is instrumental in predictive modeling, a technique that uses historical data to make predictions about future events or trends. ML algorithms can automatically identify patterns and relationships within data, enabling the creation of models that forecast future outcomes. These predictions are not based on explicit programming but on the algorithms' ability to learn from data. Businesses can use predictive modeling for a wide range of applications, such as demand forecasting, stock price prediction, and customer churn analysis.
Clustering and Classification: ML algorithms are adept at categorizing data into meaningful groups or classes, a process known as clustering and classification. This helps uncover hidden structures within the data and enables businesses to make sense of complex datasets. For instance, in customer segmentation, ML can group customers into clusters based on their behavior and preferences. In fraud detection, it can classify transactions as either legitimate or fraudulent. These applications are critical for targeted marketing, fraud prevention, and many other fields.
Anomaly Detection: Anomaly detection is another key application of Machine Learning in advanced data analysis. ML models can quickly identify unusual patterns or outliers within datasets. This is vital for various domains, including cybersecurity, quality control, and system monitoring. For instance, in cybersecurity, ML can detect unusual network activities or suspicious user behavior, allowing organizations to respond to potential threats promptly.
Regression Analysis: Regression analysis, a statistical method used for modeling the relationship between a dependent variable and one or more independent variables, is enhanced by Machine Learning. ML algorithms can handle complex, nonlinear relationships in data, allowing for more accurate and flexible modeling. This is valuable in fields like economics, epidemiology, and environmental science, where understanding the relationships between variables is critical.
Natural Language Processing (NLP) in Data Analysis
Natural Language Processing (NLP) is a pivotal aspect of data analysis that focuses on the interaction between computers and human language. In the context of data analysis, NLP serves as a bridge, enabling machines to understand, interpret, and derive insights from textual data. This includes a wide array of unstructured data sources such as articles, social media posts, customer reviews, and more.
One of the key applications of NLP in data analysis is sentiment analysis. NLP algorithms can analyze the sentiment expressed in text, helping businesses gauge the opinions and emotions conveyed in customer reviews, social media discussions, or other textual data. This is invaluable for companies seeking to understand how their brand is perceived in the market, allowing them to respond to customer sentiments in a timely and targeted manner.
Text mining is another area where NLP plays a crucial role. By extracting meaningful information from large volumes of text, NLP algorithms contribute to uncovering patterns, trends, and insights that may be hidden within textual data. This is particularly relevant in research, where vast amounts of literature and documentation can be efficiently analyzed to identify emerging themes or gain a deeper understanding of a particular subject.
The ability of NLP to process and understand human language also facilitates more natural human-computer interactions. This is evident in chatbots and virtual assistants that utilize NLP to comprehend user queries and respond in a way that mirrors human conversation. In the realm of data analysis, this means that analysts and decision-makers can interact with data more intuitively and conversationally, asking complex questions and receiving insights in a language they understand.
AI and Big Data Synergy
The synergy between Artificial Intelligence (AI) and Big Data is a dynamic partnership that has reshaped the landscape of data analysis. Big Data, characterized by its massive volume, high velocity, and diverse variety, presents a unique set of challenges that traditional data analysis methods struggle to address. Enter AI, with its ability to harness the potential of Big Data, offering a transformative solution to extract intelligent insights from the vast ocean of information.
One key aspect of this synergy is scalability. AI algorithms possess the inherent capability to scale seamlessly to the demands of massive datasets. Whether dealing with terabytes or petabytes of information, AI algorithms can process and analyze data with efficiency and speed, ensuring that valuable insights are derived promptly. This scalability is particularly crucial in industries such as finance, healthcare, and scientific research, where the sheer volume of data requires advanced computational power.
Real-time analysis is another facet of the AI and Big Data partnership that revolutionizes decision-making processes. In scenarios where time is of the essence, such as financial trading or healthcare monitoring, AI's rapid processing capabilities enable real-time analysis. This ensures that decisions are based on the most current information, facilitating quicker responses to emerging trends, threats, or opportunities.
Ethical Considerations and Bias Mitigation
In the vast landscape of artificial intelligence (AI), the ethical implications of its applications, especially in advanced data analysis, are paramount. As AI algorithms increasingly influence decision-making processes, concerns regarding bias, fairness, and transparency come to the forefront. Ethical considerations in AI encompass a spectrum of issues, including privacy concerns, unintended consequences, and the socio-economic impact of automated decision-making.
One of the critical challenges in AI is the potential for bias within machine learning models. Bias can emerge from biased training data, reflecting historical prejudices or systemic inequalities. For instance, if a facial recognition system is trained predominantly on data from a certain demographic group, it may exhibit inaccuracies when applied to other groups, potentially leading to discriminatory outcomes. Recognizing and addressing such biases is a crucial step in ensuring that AI technologies are fair and just.
Mitigating bias in AI involves a multi-faceted approach. Firstly, there's a need for diverse and representative datasets. Ensuring that the data used to train AI models is comprehensive and inclusive helps reduce biases stemming from underrepresentation. Additionally, ongoing monitoring and auditing of AI systems are essential to identify and rectify biases that may emerge over time. The transparency of AI algorithms is another key aspect; understanding how a decision is reached is vital for accountability and trust.
In the era of big data, AI stands as a beacon of innovation in the field of advanced data analysis. Its ability to learn, adapt, and uncover intricate patterns within vast datasets positions AI as an indispensable tool for businesses and researchers alike. However, as we embrace this transformative technology, it is crucial to approach it with a keen awareness of ethical considerations, ensuring that the power of AI is harnessed responsibly for the betterment of society. The journey into AI-driven data analysis is not just a technological leap; it is a commitment to shaping a future where data becomes a powerful ally in our pursuit of knowledge and progress.