AI features-Latest technological advancements
Explore the cutting-edge world of AI features with our in-depth coverage of the latest technological advancements. Stay informed about the most innovative artificial intelligence capabilities shaping the future.
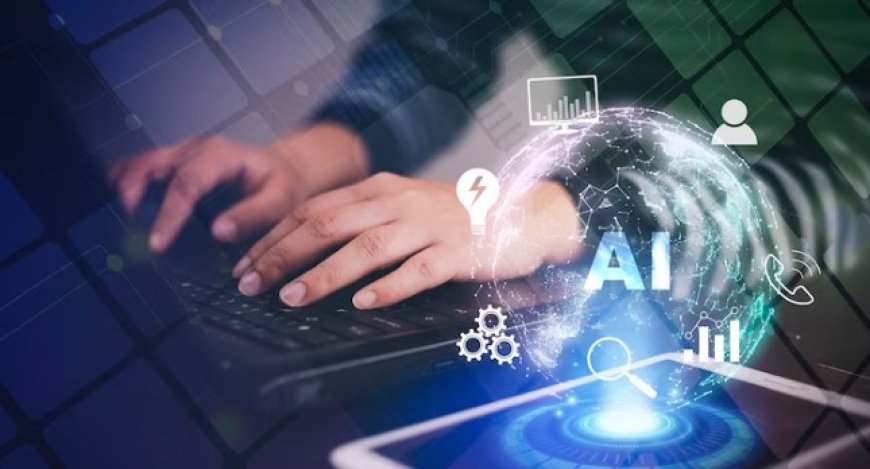
In examining the current state of AI technology, it is evident that we stand at the forefront of a transformative era. AI, once a concept confined to science fiction, has become an integral part of our daily lives. As of now, AI is not just a standalone technology but a dynamic field continuously evolving to meet the demands of our rapidly advancing society.
The role of AI features in this progression is paramount. These features, encompassing a range of capabilities such as natural language processing, machine learning, and computer vision, act as the driving force behind technological advancements. They form the core components that enable AI systems to comprehend, learn, and interact with their environment in ways that were previously unimaginable.
AI features serve as the building blocks of innovation, allowing for the creation of intelligent systems that can analyze vast datasets, recognize patterns, and make decisions with increasing accuracy. The integration of these features into various applications is not just a technological milestone but a catalyst for the next wave of progress, promising a future where AI becomes an even more integral and seamless part of our daily experiences.
The rapid evolution of AI trends
Artificial intelligence (AI) features are experiencing a rapid evolution, marked by dynamic trends in development and notable breakthroughs in recent technological advancements.
Current Trends in AI Feature Development
The prevailing trends in AI feature development reveal a strong emphasis on enhancing user experiences and system capabilities. Natural Language Processing (NLP) has emerged as a focal point, allowing machines to understand and respond to human language more intuitively. Additionally, machine learning algorithms are increasingly emphasizing interpretability and explainability, addressing concerns about the "black box" nature of some AI models.
Notable Breakthroughs in Recent Technological Advancements
Recent breakthroughs in AI have accelerated the field into uncharted territories. Advanced computer vision capabilities, for instance, enable machines to perceive and interpret visual information with unmatched accuracy. The integration of reinforcement learning has empowered AI systems to learn and adapt through trial and error, mimicking human decision-making processes.
These breakthroughs extend beyond mere technological achievements; they signify a pattern shift in how AI features are designed and deployed. Getting around this evolution requires staying in a row of emerging trends and understanding the transformative potential these breakthroughs bring to AI applications across diverse industries. The rapid evolution of AI features reflects an exciting journey toward more sophisticated, adaptable, and user-friendly AI systems.
Common hurdles faced in implementing cutting-edge AI features
1. Data Quality and Availability
-
Ensuring that the data required for training and fine-tuning AI models is of high quality can be challenging.
-
Limited availability of diverse and representative datasets can inhibit the effectiveness of cutting-edge features.
2. Computational Resources:
-
The advanced nature of certain AI features often demands significant computational power.
-
Securing the necessary infrastructure for training and deploying models can be a hold-up, especially for smaller organizations.
3. Interpretable Models:
-
As AI becomes more sophisticated, the interpretability of models becomes a challenge.
-
Understanding the decisions made by complex models is crucial, especially in fields like healthcare and finance where transparency is paramount.
4. Ethical Considerations:
-
Implementing AI features requires careful consideration of ethical implications.
-
Ensuring fairness, avoiding biases, and addressing potential ethical concerns are ongoing challenges in the development and deployment of cutting-edge features.
5. Integration with Existing Systems:
-
Incorporating advanced AI features into existing infrastructure can be complex.
-
Compatibility issues and the need for seamless integration with legacy systems pose significant hurdles.
Overcoming these hurdles is essential to fully leverage the benefits of cutting-edge AI features and ensure their successful integration into various applications and industries.
The impact of these challenges on widespread adoption
The challenges associated with implementing advanced AI features have significant consequences on their widespread adoption. These challenges encompass complexities in integration, resource-intensive requirements, ethical and regulatory considerations, skill gaps, and the need for interdisciplinary collaboration. The intricate nature of implementing these features can slow down seamless adoption, particularly for smaller organizations with limited resources.
What specific AI features are currently at the forefront of technological advancements, and how do these innovations address existing limitations and challenges within the field? In exploring the latest developments in AI features, Let’s discuss the cutting edge of technology, examining the unique capabilities that have emerged
Latest AI features
-
Natural Language Processing (NLP): NLP advancements enable AI systems to understand and interpret human language more effectively.
-
Computer Vision Enhancements: Advanced computer vision features empower AI to interpret and make sense of visual data.
-
Reinforcement Learning Innovations: Reinforcement learning is evolving with improvements in decision-making processes.
-
Explainable AI (XAI): XAI features focus on making AI algorithms more transparent and understandable
-
Automated Machine Learning (AutoML): AutoML simplifies the machine learning process, allowing non-experts to build and deploy models.
-
Generative Adversarial Networks (GANs): GANs facilitate the creation of realistic synthetic data, enhancing AI training processes.
-
Edge AI Advancements: AI capabilities are moving closer to the source of data with advancements in edge computing.
-
AI for Cybersecurity: AI features are being employed to bolster cybersecurity measures that include advanced threat detection, anomaly recognition, and proactive defense mechanisms.
-
Personalization through AI: AI is increasingly used for personalized user experiences which involves tailoring content, recommendations, and services based on individual preferences.
-
Quantum Computing Integration: Explorations into integrating AI with quantum computing are at the forefront, holding the potential to exponentially increase computational power, opening new frontiers for AI applications.
Several ways that AI features address existing limitations and challenges
Enhanced Efficiency:
New AI features often focus on improving efficiency, streamlining processes, and reducing the time required for tasks. This directly addresses the challenge of optimizing performance in complex computing tasks.
Increased Accuracy:
Advancements aim to enhance the accuracy of AI models, minimizing errors and inaccuracies in predictions or classifications. This addresses the challenge of reliability and precision in AI-driven decision-making.
Adaptability to Diverse Data Sets:
Innovations in AI features include improved adaptability to diverse data sets, overcoming challenges related to biases and limitations in handling varied information sources. This ensures more comprehensive and unbiased outcomes.
Interpretability and Explainability:
Many new AI features prioritize interpretability and explainability, addressing the challenge of the "black box" problem. This allows users to understand and trust AI decisions, particularly in critical applications like healthcare or finance.
Scalability:
Scalability is a key consideration, with advancements designed to handle increasing data volumes and complexities. This addresses challenges associated with the scalability of AI systems as they are applied to larger datasets or more extensive applications.
User-Friendly Interfaces:
Some innovations focus on user-friendly interfaces, making AI tools more accessible to individuals with diverse backgrounds. This addresses the challenge of democratizing AI usage and ensuring broader adoption across different industries.
The advancements in AI features hold the promise of transformative impacts across various industries and daily life. From revolutionizing healthcare diagnostics to optimizing manufacturing processes, the potential applications are vast. These innovations can lead to increased efficiency, better decision-making, and enhanced user experiences. In daily life, we can anticipate more personalized and intelligent applications, ranging from virtual assistants to smart homes. However, careful consideration of ethical and societal implications is paramount to ensure that the integration of these AI features aligns with human values and contributes positively to the betterment of society as a whole. As we take-up these technological strides, a thoughtful approach to their deployment will be instrumental in controlling the full potential of AI for the benefit of diverse industries and the everyday lives of individuals.